Machine Learning Is Bringing the Cosmos Into Focus
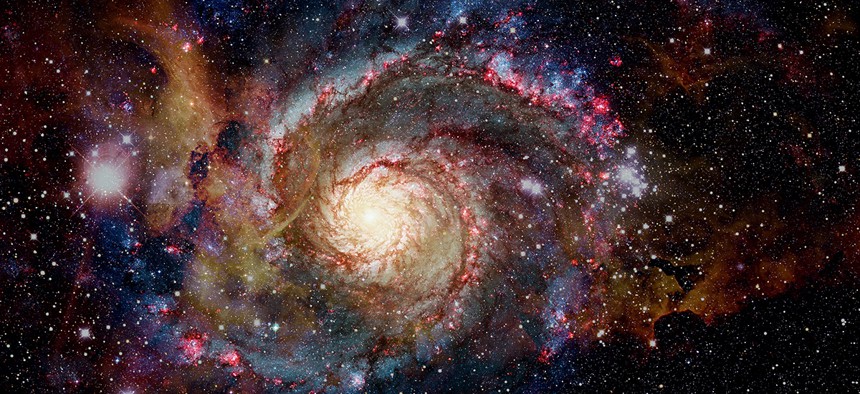
NASA images/Shutterstock.com
Training neural networks to identify galaxies could forever change humanity’s perspective of the universe.
The telescope offers one of the most seductive suggestions a technological object can carry: the idea that humans might pick up a thing, peer into it, and finally solve the riddle of the heavens as a result.
Unraveling that mystery requires its own kind of refraction in perspective, collapsing the distance between near and far as a way to understand our planet and its place in the universe.
This is why early astronomers didn’t just gaze up each night to produce detailed sketches of celestial bodies. They also tracked the movement of those bodies across the sky over time. They developed an understanding of Earth’s movement as a result. But to do that, they had to collect loads of data.
It makes sense, then, that computers would be such useful tools in modern astronomy. Computers help us program rocket launches and develop models for flight missions, but they also analyze deep wells of information from distant worlds. Ever larger telescopes have illuminated more about the depths of the universe than the earliest astronomers ever could have dreamed.
Spain’s Gran Telescopio Canarias, in the Canary Islands, is the largest telescope on Earth. It has a lens diameter of 34 feet. The Thirty Meter Telescope planned for Hawaii, if it is built, will be nearly three times larger. With telescopes, the bigger the lens, the farther you can see. But soon, artificial intelligence may help bypass size constraints and tell us what we’re looking at in outer space—even when it looks, to a telescope, like an indeterminate blob.
The idea is to train a neural network so that it can look at a blurry image of space, then accurately reconstruct features of distant galaxies that a telescope could not resolve on its own.
In a paper published in January by the Monthly Notices of the Royal Astronomical Society, a team of researchers led by Kevin Schawinski, an astrophysicist at ETH Zurich, described their successful attempts to do just that. The researchers say they were able to train a neural network to identify galaxies, then, based on what it had learned, sharpen a blurry image of a galaxy into a focused view. They used machine-learning technique known as “generative adversarial network,” which involves having two neural nets compete against one another.
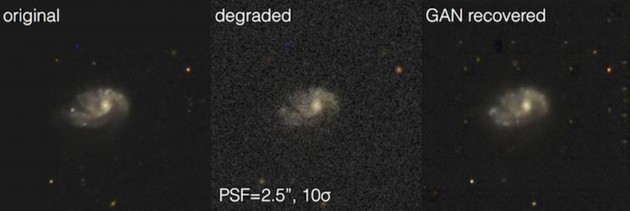
As computer scientists and physicists experiment with these techniques, increasingly powerful telescopes will offer more opportunities for neural nets to offer clarifying views of the universe. One example is the James Webb Space Telescope, or JWST, which is set to launch next year. If all goes well, the telescope will provide views of some of the oldest galaxies in the universe—ones that formed just a few hundred million years after the Big Bang. “Even JWST will have trouble to resolve these baby Milky Ways,” Schawinski told me. “A neural net might help us make sense of these images.”
“There’s a catch, though,” Schawinski told me in an email. The neural net is trained to recognize galaxies based on what we know them to be like today. Meaning, to train a neural network how to reconstruct a baby Milky Way, scientists have to be able to tell the machine what that galaxy looked like in the first place. “Now, we know that galaxies in the early universe were very different that the ones in our old, evolved universe,” Schawinski said. “So we might be training the neural net with the wrong galaxies. That’s why we have to be extremely careful in interpreting what a neural net recovers.”
It’s a crucial caveat that will continue to come up as machine learning expands across disciplines and attempts more complex applications. Elsewhere, for instance, academics have proposed using machine learning to identify the subtle signatures of a phase of matter, then reverse-engineer what it learned to generate glimpses of new materials or phases of matter, as the quantum physicist Roger Melko wrote in a recent essay.
“If we are careful enough and do this right, [using] a neural network might not be too different from what we are currently doing with more classic statistical approaches,” said Ce Zhang, a computer scientist at ETH Zurich and a co-author of the recent RAS study.
And though these efforts seem likely expand the way humans think about our place in space, humankind isn’t fundamentally changing the way it examines the universe. “None of the images from space objects, from galaxies to planets, are any more or less ‘real’ than what you might see with a human eye,” Schawinski said. “Our biological eyes can see neither X-rays nor infrared radiation, let alone focus on light from just a single transition in a particular ion.”
Imaging data is always processed in some way, he added. Human vision is its own kind of filter, and the whole history of astronomy is the story of its augmentation. “I see neural nets as the latest in sophisticated methods in telling us what’s actually there in the universe and what it means.”