More Than 50 Experts Just Told DHS That Using AI for “Extreme Vetting” Is Dangerously Misguided
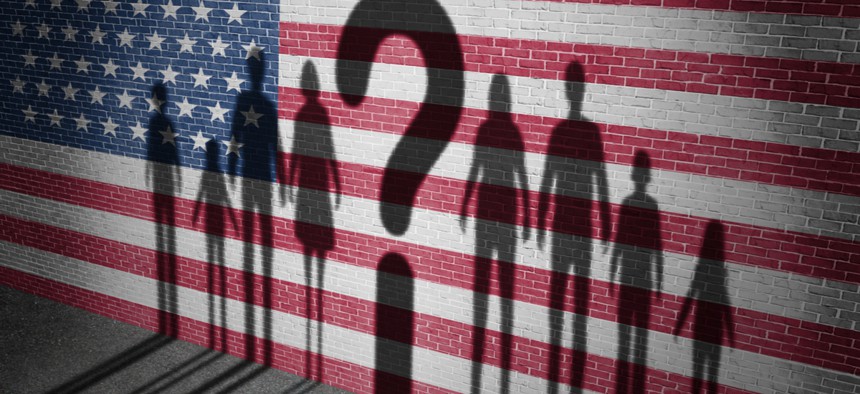
Lightspring/Shutterstock.com
The controversial program, which hasn’t yet been implemented, would screen digital information to determine whether a person is fit to stay in the United States.
A group of experts from Google, Microsoft, MIT, NYU, Stanford, Spotify, and AI Now are urging the Department of Homeland Security to reconsider using automated software powered by machine learning to vet immigrants and visitors trying to enter the United States.
The controversial program, which hasn’t yet been implemented, would screen social media posts and other digital information to determine whether the person is a “positively contributing member of society,” and if they pose a threat to the United States. The process, called extreme vetting, was spurred by a June executive order from President Trump. However, these 54 experts say those metrics are impossible to determine using any machine learning or automated approach available today or foreseeable in the future.
No data or measures exist that could determine if someone is a good citizen. Thus the DHS algorithms have nothing to learn from.
“Because these characteristics are difficult (if not impossible) to define and measure, any algorithm will depend on ‘proxies’ that are more easily observed and may bear little or no relationship to the characteristics of interest,” the letter reads.
These proxies, like the amount of money someone makes or whether they have a common job, could easily stand in for positive contributions to society, which could in turn lead people who are poor being turned away in greater numbers.
“Algorithms designed to predict these undefined qualities could be used to arbitrarily flag groups of immigrants under a veneer of objectivity,” the experts write.
Notably not on the list of signees is a representative from IBM, which expressed interest in building the DHS software at a conference in July, according to a report by The Intercept.
NEXT STORY: Your Boss Might Be Better as an Algorithm