Stanford Trained AI to Diagnose Pneumonia Better Than a Radiologist in Just Two Months
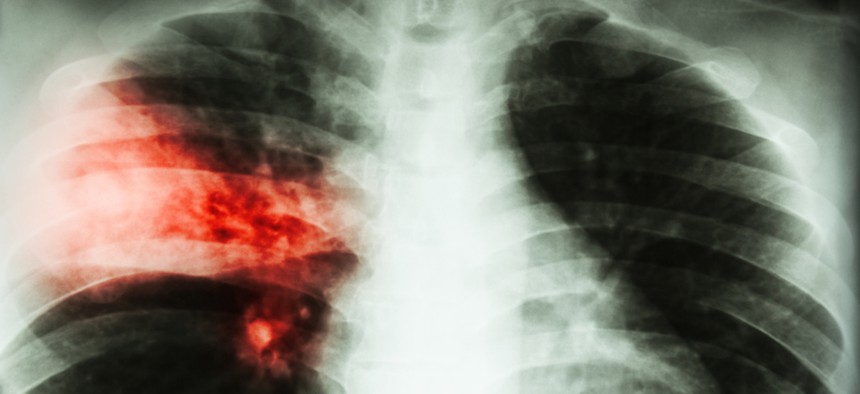
Puwadol Jaturawutthichai/Shutterstock.com
There’s a clear trend that having more data makes it easier to train artificial intelligence.
There’s a clear trend that having more data makes it easier to train artificial intelligence. Bigger datasets, like ImageNet, originally showed that AI could be useful for tasks like image recognition, leading to a race among everyone from large technology companies to academics to compile new datasets to stretch the limits of AI.
Now, a new paper from Stanford University shows just how fast a new dataset could be used to train artificial intelligence algorithms to the point of near-human accuracy. Using 100,000 x-ray images released by the National Institutes of Health on Sept. 27, the research published Nov. 14 (without peer review) on the website ArXiv claims its AI can detect pneumonia from x-rays with similar accuracy to four trained radiologists.

That’s not all—the AI was trained to analyze x-rays for 14 diseases NIH included in the dataset, including fibrosis, hernias, and cell masses. The AI’s results for each of the 14 diseases had fewer false positives and false negatives than the benchmark research from the NIH team that was released with the data.
The paper includes Google Brain founder Andrew Ng as a co-author, who also served as chief scientist at Baidu and recently founded Deeplearning.ai. He’s often been publicly bullish on AI’s use in healthcare.
“I think health care 10 years from now will use a lot more AI and will look very different than it does today,” he told MIT Tech Review earlier this year.
These algorithms will undoubtedly get better—accuracy on the ImageNet challenge rose from 75% to 95% in just five years—but this research shows the speed at which these systems are built is increasing as well.