What Your Boss Could Learn by Reading the Whole Company’s Emails
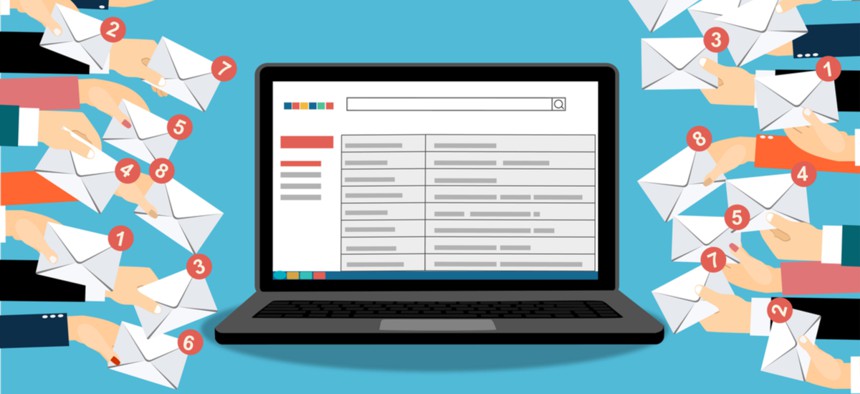
Tetiana Yurchenko/Shutterstock.com
Employee emails contain valuable insights into company morale—and might even serve as an early-warning system for uncovering malfeasance.
When Andrew Fastow, the former chief financial officer of Enron, finishes a public-speaking gig these days, a dozen or so people from the audience are typically waiting to talk to him. Some ask about his role in the scandal that brought down the energy company. Others want to know about his six years in prison. After a 2016 event in Amsterdam, as the crowd was thinning out, Fastow spotted two men standing in a corner. Once everyone else had left, they walked up to him and handed him a laminated chart.
The men were there on behalf of KeenCorp, a data-analytics firm. Companies hire KeenCorp to analyze their employees’ emails. KeenCorp doesn’t read the emails, exactly—its software focuses on word patterns and their context. The software then assigns the body of messages a numerical index that purports to measure the level of employee “engagement.” When workers are feeling positive and engaged, the number is high; when they are disengaged or expressing negative emotions like tension, the number is low.
The two men in Amsterdam told Fastow that they had tested the software using several years’ worth of emails sent by Enron’s top 150 executives, which had become publicly available after the company’s demise. They were checking to see how key moments in the company’s tumultuous collapse would register on the KeenCorp index. But something appeared to have gone wrong.
The software had returned the lowest index score at the end of 2001, when Enron filed for bankruptcy. That made sense: Enron executives would have been growing more agitated as the company neared insolvency. But the index had also plummeted more than two years earlier. The two men had scoured various books and reports on Enron’s downfall, but it wasn’t clear what made this earlier date important. Pointing to the sudden dip on the left side of the laminated chart, they told Fastow they had one question: “Do you remember anything unusual happening at Enron on June 28, 1999?”
The so-called text-analytics industry is booming. The technology has been around for a while—it powers, among other things, the spam filter you rely on to keep your inbox manageable—but as the tools have grown in sophistication, so have their uses. Many brands, for instance, rely on text-analytics firms to monitor their reputation on social media, in online reviews, and elsewhere on the web.
Text analytics has become especially popular in finance. Investment banks and hedge funds scour public filings, corporate press releases, and statements by executives to find slight changes in language that might indicate whether a company’s stock price is likely to go up or down; Goldman Sachs calls this kind of natural-language processing “a critical tool for tomorrow’s investors.” Specialty-research firms use artificial-intelligence algorithms to derive insights from earnings-call transcripts, broker research, and news stories.
Does text analytics work? In a recent paper, researchers at Harvard Business School and the University of Illinois at Chicago found that a company’s stock price declines significantly in the months after the company subtly changes descriptions of certain risks. Computer algorithms can spot such changes quickly, even in lengthy filings, a feat that is beyond the capacity of most human investors. The researchers cited as an example NetApp, a data-management firm in Silicon Valley. NetApp’s 2010 annual report stated: “The failure to comply with U.S. government regulatory requirements could subject us to fines and other penalties.” Addressing the same concern in the 2011 report, the company clarified that “failure to comply” applied to “us or our reseller partners.” Even a savvy human stock analyst might have missed that phrase, but the researchers’ algorithms set off an alarm.
Granted, the study scoured old filings; the researchers had the benefit of hindsight. Still, a skeptical investor, armed with the knowledge that NetApp had seen fit to make this change, might have asked herself why. If she’d turned up an answer, or even just found the change worrying enough to sell her stock, she’d have saved a fortune: Embedded in that small edit was an early warning. Six months after the 2011 report appeared, news broke that the Syrian government had purchased NetApp equipment through an Italian reseller and used that equipment to spy on its citizens. By then, NetApp’s stock price had already dropped 20 percent.
While text analytics has become common on Wall Street, it has not yet been widely used to assess the words written by employees at work. Many firms are sensitive about intruding too much on privacy, though courts have held that employees have virtually no expectation of privacy at work, particularly if they’ve been given notice that their correspondence may be monitored. Yet as language analytics improves, companies may have a hard time resisting the urge to mine employee information.
One obvious application of language analysis is as a tool for human-resources departments. HR teams have their own, old-fashioned ways of keeping tabs on employee morale, but people aren’t necessarily honest when asked about their work, even in anonymous surveys. Our grammar, syntax, and word choices might betray more about how we really feel.
Take Vibe, a program that searches through keywords and emoji in messages sent on Slack, the workplace-communication app. The algorithm reports in real time on whether a team is feeling disappointed, disapproving, happy, irritated, or stressed. Frederic Peyrot, one of Vibe’s creators, told me Vibe was more an experiment than a product, but some 500 companies have tried it.
Keeping tabs on employee happiness is crucial to running a successful business. But counting emoji is unlikely to prevent the next Enron. Does KeenCorp really have the ability to uncover malfeasance through text analysis?
That question brings us back to June 28, 1999. The two men from KeenCorp didn’t realize it, but their algorithm had, in fact, spotted one of the most important inflection points in Enron’s history. Fastow told me that on that date, the company’s board had spent hours discussing a novel proposal called “LJM,” which involved a series of complex and dubious transactions that would hide some of Enron’s poorly performing assets and bolster its financial statements. Ultimately, when discovered, LJM contributed to the firm’s undoing.
According to Fastow, Enron’s employees didn’t formally challenge LJM. No one went to the board and said, “This is wrong; we shouldn’t do it.” But KeenCorp says its algorithm detected tension at the company starting with the first LJM deals.
Today, KeenCorp has 15 employees, half a dozen major clients, and several consultants and advisers—including Andy Fastow, who told me he had been so impressed with the algorithm’s ability to spot employees’ concerns about LJM that he’d decided to become an investor. Fastow knows he’s stuck with a legacy of unethical and illegal behavior from his time at Enron. He says he hopes that, in making companies aware of KeenCorp’s software, he can help “prevent similar situations from occurring in the future.”
I was skeptical about KeenCorp at first. Text analysis after the fact was one thing, but could an analysis of employee emails actually contain enough information to help executives spot serious trouble in real time? As evidence that it can, KeenCorp points to the “heat maps” of employee engagement that its software creates. KeenCorp says the maps have helped companies identify potential problems in the workplace, including audit-related concerns that accountants failed to flag. The software merely provides a warning, of course—it isn’t trained in the Sarbanes-Oxley Act. But a warning could be enough to help uncover serious problems.
Such early tips might also become an important tool to help companies ensure that they are complying with government rules—a Herculean task for firms in highly regulated fields like finance, health care, insurance, and pharmaceuticals. An early-warning system, though, is only as good as the people using it. Someone at the company, high or low, has to be willing to say something when the heat map turns red—and others have to listen. It is hard to imagine Enron’s directors heeding any warning about the use of complex financial transactions in 1999—the bad actors included the CEO, and we know that whistle-blowers at the company were ignored.
The potential benefits of analyzing employee correspondence must also be weighed against the costs: In some industries, like finance, the rank and file are acutely aware that everything they say in an email can be read by a higher-up, but in other industries the scanning of emails, however anonymous, will be viewed as intrusive if not downright Big Brotherly.
But it is managers who might have the most to fear from text-analysis tools. Viktor Mirovic, KeenCorp’s CFO, told me that the firm’s software can chart how employees react when a leader is hired or promoted. And one KeenCorp client, he said, investigated a branch office after its heat map suddenly started glowing and found that the head of the office had begun an affair with a subordinate.
When I asked Mirovic about privacy concerns, he said that KeenCorp does not collect, store, or report any information at the individual level. According to KeenCorp, all messages are “stripped and treated so that the privacy of individual employees is fully protected.” Nevertheless, Mirovic concedes that many companies do want to obtain information about individuals. Those seeking that information might turn to other software, or build their own data-mining system.
Text analysis is a fledgling technology. It remains unclear how often such tools might suggest a problem when none exists, and not all wrongdoing will register on a heat map, no matter how finely tuned.
Still, a market will surely emerge for services claiming that they can find useful information in our work emails. Adam Badawi, a colleague of mine at UC Berkeley, uses natural-language algorithms to assess regulatory filings. He predicts that text analytics will become part of legal-and-compliance culture as the tools grow more sophisticated. Firms will want to protect themselves from liability by examining employee communications more comprehensively, particularly with respect to allegations of bias, fraud, and harassment. “This is something companies are hungry for,” Badawi told me.
In an ideal world, employees would be honest with their bosses, and come clean about all the problems they observe at work. But in the real world, many employees worry that the messenger will be shot; their worst fears stay bottled up. Text analytics might allow firms to gain insights from their employees while intruding only minimally on their privacy. The lesson: Figure out the truth about how the workforce is feeling not by eavesdropping on the substance of what employees say, but by examining how they are saying it.