Making Algorithms Less Biased
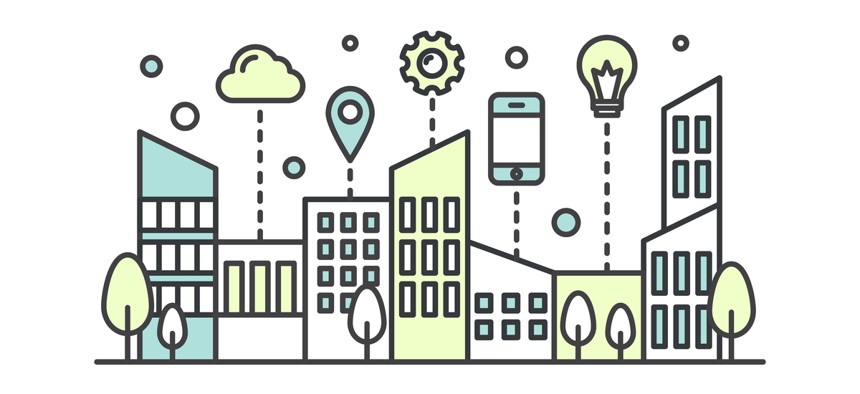
Boo-Tique/Shutterstock.com
In the smart city era, data is increasingly used in governments’ decisionmaking. But what happens when that data is flawed?
For several years now, critics have raised questions about new risk assessments used to help judges decide whether defendants can be released from jail before trial. They have argued that the algorithms at the heart of those reviews too often end up introducing racial bias into what is supposed to be a more fair process.
The Center for Government Excellence on Monday released a toolkit designed to help local officials root out that kind of bias by improving the fairness and transparency of data science projects in their pipelines.
Human review is needed to minimize unintentional harm to residents from inequitable automated decisions, Andrew Nicklin, director of data practices at GovEx, told Route Fifty. The new toolkit from the Center for Government Excellence, or GovEx, offers a risk management approach to algorithms, which are now used in many aspects of government, from criminal justice to smart cities to education.
“We focused on creating something that was practical and not oriented toward a data science expert,” Nicklin said.
The project has its roots in the stories about flawed algorithms, which relied on racially biased arrest data, being used in bail determinations or even in the sentences imposed by judges. The Johns Hopkins University-based center’s toolkit evolved out of academic and nonprofit discussions about how to fix the growing problem.
In partnership with San Francisco’s DataSF, Washington D.C.’s Data Community DC and Harvard University’s Civic Analytics Networks, GovEx began work in earnest in February on a toolkit that provides officials with questions they can ask of data science projects.
“Instead of wringing our hands about ethics and AI, our toolkit puts an approachable and feasible solution in the hands of government practitioners—something they can use immediately, without complicated policy or overhead,” said Joy Bonaguro, former chief data officer for San Francisco, in a statement.
The toolkit’s questions help officials determine their risk score—questions like whether an algorithm makes use of an old dataset that’s survived several cultural and organizational changes. That could leave the algorithm at “high risk of historical bias,” Nicklin said.
GovEx collapses risk factors down into seven categories like “accountability risk,” which asks who or what is making decisions, how they are made, if they can be explained, and if there is a review process in place.
An algorithm that automatically makes decisions is characteristic of smart city projects but much higher risk than one that simply presents information. Algorithms that don’t involve machine learning are much easier to explain than those employing complex data science that even the designers don’t fully comprehend the implications of, Nicklin said.
“Sometimes you’re going to have to be able to say, ‘Here’s why an algorithm made a decision,’” he added.
Audits may also be necessary to reproduce the conditions that created a result or tweak the algorithm, which means algorithms where the code can’t be accessed are riskier, Nicklin said.
At Bloomberg’s Data for Good Exchange conference over the weekend, New York City tested the toolkit out on the automation behind its teacher evaluations, while other localities evaluated their algorithms for determining whether a child is at risk of abuse or neglect.
On the mitigation side of the equation, GovEx’s toolkit also offers strategies for reducing risk.
Data science projects might require a performance monitoring program or large-scale review board depending on the situation.
“In some cases that corrective action might be: This project may be too risky,” Nicklin said. “You may want to consider not doing it.”