Here Come AI-Enabled Cameras Meant to Sense Crime Before It Occurs
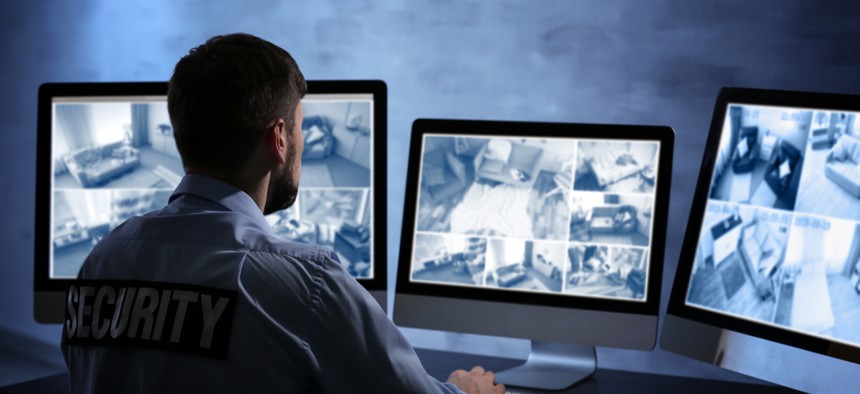
Africa Studio/Shutterstock.com
The future of video surveillance is about detecting not just faces, but behaviors.
In 1787, English philosopher Jeremy Bentham came up with an idea for a prison that would cost a fraction of the cost of other contemporary jails to run with virtually no internal crime. His theoretical prison, the panopticon, was curved, the cells facing inward toward a center point where a guard tower would stand. The windows in the guard tower were to be darkened on one side. This way, a single guard would be able to observe the behavior of all the prisoners. But more importantly, the prisoners would never know whether the guard had his or her gaze trained on them. The end result, every individual within the prison internalizes a sense of being watched all the time and behaves accordingly.
This idea of the panopticon has become a stand-in for the threat of ubiquitous surveillance, due mostly to Bentham’s choice of setting—a prison. But Bentham aimed not to frighten people, but to furnish a way to manage a scarce resource: the attention of law enforcement.
A new trend in video surveillance technology is turning Bentham’s panopticon into reality, but not in the way he imagined. Instead of a prison, the new panopticon would focus the attention of law enforcement on a person when her behavior becomes relevant to the guard tower. Imagine it were possible to recognize not the faces of people who had already committed crimes, but the behaviors indicating a crime that was about to occur.
Multiple vendors and startups attending ISC West, a recent security technology conference in Las Vegas, sought to serve a growing market for surveillance equipment and software that can find concealed guns, read license plates and other indicators of identity, and even decode human behavior.
A company called ZeroEyes out of Philadelphia markets a system to police departments that can detect when a person is entering a given facility carrying a gun. It integrates with any number of closed-circuit surveillance systems. But machine learning algorithms don’t just come out of a box knowing how to recognize a firearm any more than a drug dog arrives from the breeder knowing the difference between marijuana and oregano. To teach the algorithm, a team from the company shows up on location and proceeds to stage mock attacks. Slowly, the algorithm begins to learn what a gun looks like in that specific setting, depending on light, angles, and other conditions. They’re currently working with New York City Schools and have a contract with U.S. Customs and Border Patrol, but are not yet deployed to the border, said Kenny Gregory, a software engineer at the company.
Automated firearm detection is one solution to a growing problem that has no clear policy cure: curbing mass shootings and gun violence. While some polls show that 70 percent of Americans support stricter gun laws, that number is far lower, about 31 percent, among conservatives. And so the political debate, while fiery, has stalled. Gun-detection algorithms that alert security personnel when an armed person arrives might reduce the number of victims — though likely not as much as if there were no armed shooter in the first place.
It’s a predictive indicator of potential violence, rather than a lagging indicator, such as facial recognition, and carries less political baggage. More and more, cities and police departments are experimenting with facial recognition to detect the presence of suspects in real time. They’re meeting with stiff resistance from privacy advocates in San Francisco, where some lawmakers are looking to block deployment, and elsewhere.
But the two indicators—the identity of someone in a criminal database, the presence of a weapon in the hand of that person—together would provide a far more accurate probability and cut down on false positives. In lieu of facial recognition, some police departments may rely on automated license plate readers as an indicator of identity. A company called Reconyx sells a system that can automatically read license plates and has multiple law enforcement clients. Automated license-plate reading is an issue where privacy advocates convinced a Virginia judge to limit its use among police departments.
But policy efforts to rein in the use of artificial intelligence in surveillance move much slower than the technology itself.
Machine recognition of faces or license plates is a subject of privacy concern because these speak to identity. But any number of data streams may speak to the same thing They include biometric data such as gait or speech, which may be accessible via video streams, or even location patterns, which are detectable via cell phone. It’s one reason why you might see a whole different set of advertisements depending on where you are. Cellular providers and others nominally “anonymize” this data before sending it to third-party marketers, but research shows that anonymization techniques can rather easily be reversed by comparing multiple datasets.
The presence of a specific object, like a gun, with a specific person, like someone in a criminal database, serves as an imperfect indicator of violence. The future of predicting violence may rest in the collection of other data related to behavior. This is where a Peter Thiel-backed startup called Athena Security is distinguishing itself among companies that do object and facial recognition.
Athena sells software that can recognize specific behaviors, like fighting, walking slowly when others are walking fast, virtually anything that aberrates from the norm.
The software can detect that a fight is about to occur milliseconds after the first punch is launched, before it even lands on the victim. “We’re measuring the velocity of a punch,” said company founder and chief technical officer Chris Ciabarra.
Athena can combine that with other data points, such as the facial expression of the participants in the interaction, to determine mood, even intent.
“With behavior, we’re looking at the emotions on their face. Are they angry? Are they sad?” he said.
But Athena also does object recognition, such as finding guns. They say that they don’t need to do on-site model development. The system works similar to the way the brain does. When it detects an anomaly, it focuses on it, then reweighs the data to reach a higher level of confidence. “As soon as we think we’ve found a gun, we stop. It zooms into our next detailed image for the computer,” Ciabarra said. “If there’s a gun, boom, we get it. It helps get rid of all of our false positives. We train it the way a human being would look at it.”
They’re moving into thermal imaging as well, to detect concealed guns. And they’re claiming a success rate of 99 percent. The only constraint is the camera; higher-resolution cameras allow them to get farther away. But the prediction activity is the same.
Moreover, says Ciabarra, it works with virtually any behavior detectable by camera that you want to see and predict. “We have all of these customers coming to us saying, ‘I have this need, I want to figure out when someone falls down.’ We can build that out for someone,” he said.
In essence, if it’s a behavior that humans can observe, you can train a machine to observe it as well. Ciabarra says the company is talking with the U.S. Air Force and other government buyers, but is finding the most interest among Fortune 500 companies.
China is also looking to play an increasingly large role. Nearly two dozen Chinese companies were at the expo selling cameras and security software. An industry representative from the Chinese delegation, who asked to remain anonymous, said that more and more Chinese surveillance equipment manufacturers are looking to add value to their product pitch by offering artificial intelligence software for facial recognition or gun recognition.
Abnormal behavioral recognition is also an area where there is a growing body of Chinese scholarship.
There’s really no legal barrier to collecting video data on human behavior in open or public settings. Red-light cameras do so every day within the limits of a very specific behavior. But the possibility of taking in virtually all human activity that might be observed and then separating out the normal versus the abnormal creates a new dilemma for privacy advocates in the West. It’s a dilemma that will only grow if future tools actually do result in faster police reactions, potentially saving lives.
A key determining factor will be the cost of false positives. If that cost—both for the observed individual and the observer— takes the form of expensive legal cases, wrongful detention, etc., the public rejection of behavior surveillance will likely grow. If it the cost of the false positive is limited to a few questions from a human, or, perhaps just a second glance from a different piece of machine learning software, then it’s easier to imagine public acceptance of behavioral surveillance, especially as the overall false positive rate goes down.
It would seem Bentham was being too conservative when he imagined the future of surveillance. It is possible to create not just a collective feeling of always being watched, but the reality of it as well.