The U.S. Army Wants AI to Read Soldiers’ Minds
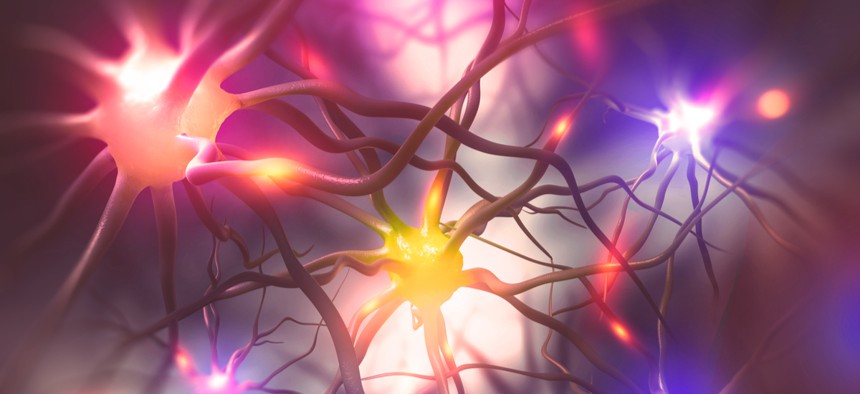
Yurchanka Siarhei/Shutterstock.com
A new study from the Army Research Lab may help AI-infused weapons and tools better understand their human operators.
In World War II, the Allies had a big problem. Germany’s new bombers moved too quickly for the anti-aircraft methods of the previous war, in which soldiers used range tables and hand calculations to line up their guns. Mathematician Norbert Wiener had a theory: the only way to defeat the German aircraft was to merge the gun and its human operators—not physically but perceptually, through instruments. As Weiner explained in the video below, that meant “either a human interpretation of the machine, or a machine interpretation of the operator, or both.” This was the only way to get the gun to fire a round on target—not where the plane was but where it was going to be. This theoretical merger of human and machine gave rise to the field of cybernetics, derived from the Greek term cyber, to steer, and the English term net, for network.
In the modern context, the merger of the human and the machine has taken on a new importance in the planning of warfare, especially as the U.S. and other militaries move forward with ever more autonomous weapons that function with the ruthless speed and efficiency of electronica but still require human supervision and control.
“Part of our vision is closing the loop between the system and the warfighter,” Mike LaFiandra, chief of the dismounted warrior branch at Army Research Laboratory, or ARL, said at a 2017 NDIA event. Let the system understand what’s happening with the warfighter, make that more a symbiotic relationship where the system is predicting based on this warfighter’s physiology, And the system knows because its been training with him for years, this is what we expect is going to happen soon and this is the specific mitigation strategy that that warfighter needs to do better.”
Army scientists this week published a new study in the journal Science Advances, showing the future of that exchange, the ability of machines to better understand their operators’ thoughts and intent based on what the operator’s brain is doing. The study explores how the brain shifts to different states, from distracted to ordered and aware, based on how different its regions are behaving and communicating with one another.
In particular, the paper looks at how the brain arrives at “chimera states,” in which several of its regions are focused on one task.
There is no good or bad chimera state, according to Jean Vettel, a co-author and senior neuroscientist at the ARL’s Combat Capabilities Development Command. But the chimera states do differ in terms of what’s optimal for different environments.
An optimal chimera state is one where the right parts of the brain are doing the right thing at the right time. Vettel compared it to when a person walks into a restaurant (the brain is the restaurant) and the hostess, the waiter, and the busboys are all focused, operating in sync, around the task of getting the person seated at a clean table, with a menu in hand, bread on the table, etc. The kitchen staff will have a role to play later and that’s when their undivided attention and focus will be important. But not at first. When things are synchronized properly, all the regions are focused on the right thing at the right time.
The work sought to show how “dynamical states give rise to variability in cognitive performance, providing the first conceptual framework to understand how chimera states may subserve human behavior.”
Understanding those alignments between the kitchen and the waitstaff and the customer is key to getting humans and computers to work together, which is critical to where the military wants human-machine teaming to go.
The goal isn’t just to understand how chimera states emerge generally, it’s to better map how they emerge in individuals since all people are different. That’s what is going to make future AI-enabled assistants effective partners, whether as a targeting aids in a tank or software to sort through hundreds of hours of video-footage and tip off a human analyst when something important shows up. These future artificial entities will need to be able to read their individual human operators in a way that machines don’t today, since no two brains synchronize regions or transition between states in exactly the same way.
Illustrating what that looks like in terms of the way humans will operate with AI-enabled software in the future requires a different metaphor.
Look at the way Gmail now offers suggestions for how you should finish a sentence when you type a new email. The suggestions are banal, deprived of uniqueness or personality. They understand human intention only in terms of an average gleaned from data from lots of people and their responses. Now imagine that Gmail was able to anticipate the most likely thing you would type, when you are at your best. That’s the goal for applying neuroscience to future human-machine teaming in the military.
Achieving that level of predictability of intent requires a lot of exposure to an individual operator’s biophysical data. That’s a key area for future research because that data is hard to get, especially in the context of a battlefield. Vettel and her colleagues’ research used MRI machines, which are too big to collect data on an individual in any situation other than a lab.
But the MRI data on transitions between brain states will become vital when smaller, better sensors allow machines to collect biophysical data without getting in the way of what the human is doing.
“Let’s assume the ability to record brain data and measure brain data has been wildly improved over what we can currently do,” Vettle said. “Our research is trying to figure out if we can link those brain signals into a better understanding of your intent.”
Right now, the work is focused on “understanding intent” seconds to minutes before the individuals’ future action. But future work could focus on understanding how loss of sleep, the presence of stress (or, conversely, the presence of sleep and the absence of stress) will affect those brain states. That could, possibly, allow the prediction of brain states far in advance. That, in turn, could revolutionize not only human and machine teaming but future training and learning in general. “If we could understand how brains are changing as expertise is acquired, then we could quantify the timescale of expertise development,” she said.
It might be possible, says Vettel, to actually know when someone has absorbed new information based on how their brain states are changing. That could enable human beings to learn far faster than they learn today… but not faster than the machines around them.