How the Bureau of Labor Statistics is Ditching Hand-coding Data
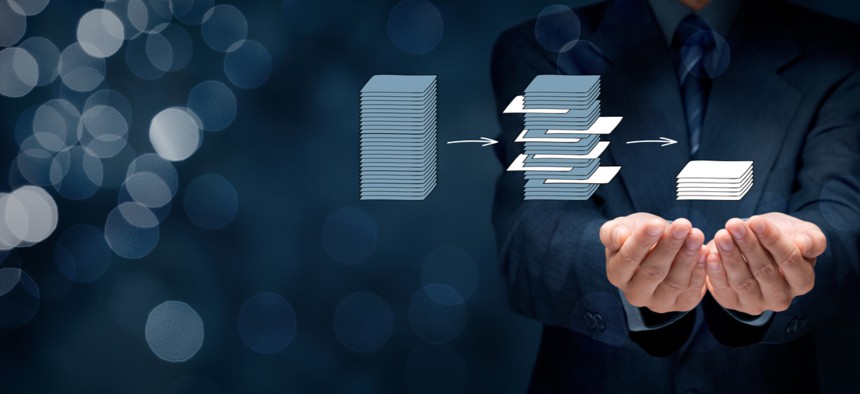
Jirsak/Shutterstock.com
Using some DIY-attitude and machine learning tools, BLS figured out how to automate 85% of its survey workload.
Implementing machine learning and artificial intelligence has radically transformed the Bureau of Labor Statistics’ productivity, freed up its workforce to perform less menial tasks and has resulted in more accurate survey analysis.
The fact-finding agency processes hundreds of thousands of surveys each year to provide the government and public with essential statistical data about society and the economy. In the past, converting the text-heavy records into different codes that make sense of the data required BLS workers to engage in tedious manual labor that didn’t always result in the most accurate outcomes. But automating the once manual processes has had lasting impacts across BLS.
“This all actually worked out much better than we expected,” Alex Measure told Nextgov. Measure was originally hired as an economist at BLS, but over the last eight years he’s led some of the agency’s efforts around integrating machine learning to complete tasks previously done by hand.
For example, each year the agency conducts the Survey of Occupational Injuries and Illnesses, which collects hundreds of thousands of written descriptions regarding work-related afflictions. In the past, Measure said humans would spend countless hours converting the key pieces of text from each survey into codes so that BLS could discern the data. The results would provide insights like how many U.S. janitors were injured on the job annually, or what the most common injuries might be.
“As you can imagine, when you are collecting about 300,000 of these each year and having people read through them by hand and code them by hand, it really adds up to being a lot of work,” he said.
The bureau began exploring different ways to use computers to automate the work.
“The idea in supervised machine learning is you take a bunch of examples of these narratives that have been coded, and then you try to get the computer to learn from these previously coded examples. Ideally, it will learn how to perform this task on its own if you give it enough examples and the right algorithm to learn,” Measure said.
His team realized early on that they could use open-source software to automate their processes and they immediately observed positive results. Not only were they able to train the original systems in just a few weeks, but the original computer-coded results turned out to be more accurate than the results from trained humans. Earlier this year, BLS started switching to “deep neural network models,” which allow for more layers of machine learning and have made “significantly fewer errors” than humans and the earlier systems.
“So the impact is that we’ve automated a very large portion of this sort of routine coding work,” Measure said. “And we are now automatically assigning roughly 85% of our primary codes using these algorithms, so that’s freed up our staff to spend more time on other important things.”
Measure said it’s also enabled BLS to enhance its results because now workers have more time to reach out to companies they need responses from and review the coded outcomes to improve the data they produce.
“So far, I think that people have seen this as a tool that helps them do their job better and I think it’s resulted in big quality improvements that have come from that,” he said.
The bureau is now applying AI to many more projects and Measure said he’s excited to watch the tech continue to evolve and for some of the latest projects in the works. He also believes BLS’ learnings are applicable for other agencies.
Because “some of the best tools” his team used were open source and available through Google and Facebook internally for free, other agencies that process big data don’t need to spend tens of thousands of dollars on proprietary software.
He also noted that websites like Coursera and edX, which he used to learn the tech, offer free or cheap training tools that anyone can adopt to master machine learning.
“I think the most interesting aspects of this to me are actually how accessible the technology is,” Measure said. “Obviously economists are not people who are trained in AI and so it was really interesting to me that I could learn these skills relatively easy and I think a lot of these skills are closely related to skills that people already have in other disciplines.”