GSA Official: Agencies Should Build Testing Data and Environments Specifically for AI
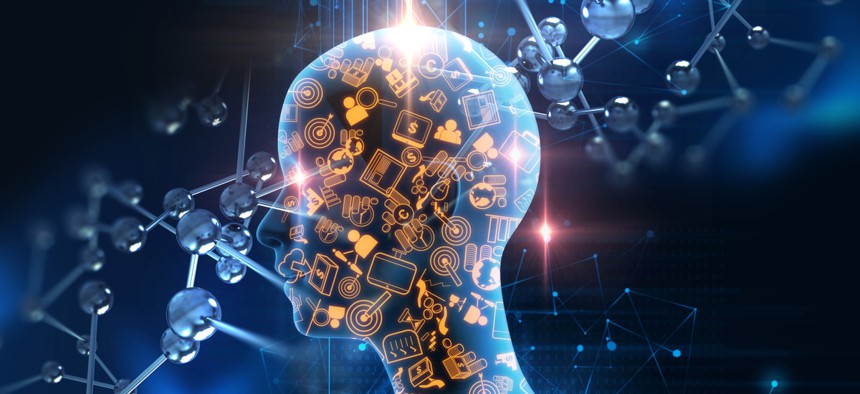
whitemocca/Shutterstock
Unless agencies start thinking differently, the security process will continue to stand in the way of new companies getting access to the real-world data required to develop artificial intelligence and machine learning tools.
For a machine-learning algorithm to be truly—and, in some cases, just marginally—effective, it needs to be trained using data sets that are as close to real-life collections as possible. However, when it comes to developing new products in tandem with the federal government, agencies are often reticent to share sensitive data with untested programs and companies.
Federal agencies are under mandate to protect the data they collect, particularly when it comes to people’s personally identifiable information or companies’ intellectual property. To ensure sensitive data isn’t lost or stolen through a third-party platform, IT products and services sold to agencies must have an authority to operate, or ATO—an often time- and money-consuming process that slows deployment but ensures a baseline of security.
But when it comes to encouraging new companies and cutting-edge products to work with the government, the ATO process has been a significant barrier, especially in machine learning and artificial intelligence, according to Chris Hamm, director of the General Services Administration’s FEDSIM, which offers acquisition assistance on highly technical procurements.
“You identified the No. 1 problem I have in interacting with the new AI and ML companies,” Hamm said Wednesday in response to a question at GSA’s AI in Federal Procurement event.
When working with companies new to the federal space, Hamm said he first tries to bring them through the ATO process. However, this has created a Catch-22 in the AI and machine learning area: The vendor needs to prove its machine-learning algorithm will meet an agency’s requirements before moving forward but can’t get access to real-world training data without an ATO.
The need for real-world training data is so acute that even the Defense Department pushes algorithms into the field in the early stages of development—something the risk-averse Pentagon wouldn’t do with, say, a weapons system.
“We don’t send weapons systems to the field at 50% effectiveness and then hope to iterate over time. We have a very rigorous process before they get cleared,” Peter Ranks, Defense deputy CIO for information enterprise, said during a keynote at the Professional Services Council’s 2019 Vision conference in October. “That does not work for AI. You cannot wait for your AI to be perfect on practice data before it’s going to go out and meet the enemy. It needs to be deployed and it needs to iterate quickly.”
Ranks said the department is working through a culture change on this issue and is now putting mid-development AI algorithms into the field “when they still have a significant amount of training where they have to encounter real users and real data in order to make them better.”
From Hamm’s perspective, the ideal solution would be something in between the real-world and synthetic data.
“We would allow everyone to use data that’s been sanitized in some way that allows us to test the algorithm that isn’t necessarily the same as the production environment but doesn’t require the entity to go through all of the security requirements,” he said, arguing that every agency would need to build its own such environment.
“I think over time there’s going to be an evolution of: there’s the existing production environment, there’s an in-between area where we do our testing and then there’s the corporate side,” he said.
Hamm acknowledged that this structure would still require some security validations but far less than a standard ATO process today.