CBP Is Upgrading to a New Facial Recognition Algorithm in March
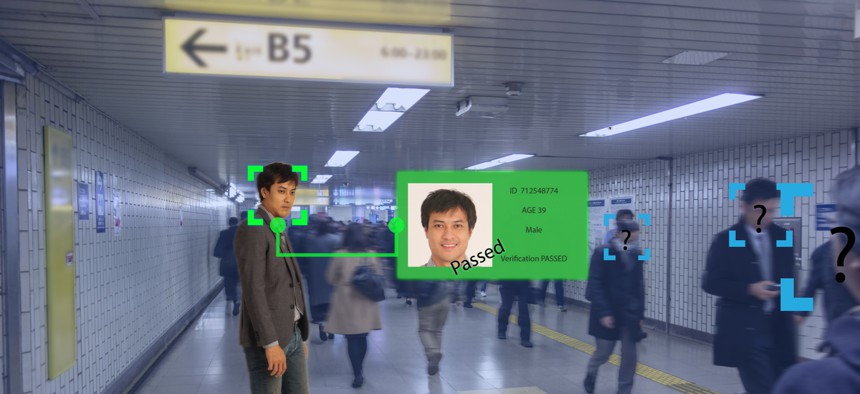
MONOPOLY919/Shutterstock.com
The agency also signed an agreement with NIST to test the algorithm and its operational environment for accuracy and potential biases.
Customs and Border Protection is getting ready to upgrade the underlying algorithm running in its facial recognition technology and will be using the latest from a company awarded the highest marks for accuracy in tests by the National Institute of Standards and Technology.
CBP and NIST also entered an agreement to conduct full operational testing of the border agency’s program, which will include a version of the algorithm that has yet to be evaluated through the standards agency’s program.
CBP has been using facial recognition technology to verify the identity of travelers at airports and some land crossings for years now, though the accuracy of the underlying algorithm has not been made public.
At a hearing Thursday of the House Committee on Homeland Security, John Wagner, CBP deputy executive assistant commissioner for the Office of Field Operations, told Congress the agency is currently using an older version of an algorithm developed by Japan-based NEC Corporation but has plans to upgrade in March.
“We are using an earlier version of NEC right now,” Wagner said. “We’re testing NEC-3 right now—which is the version that was tested [by NIST]—and our plan is to use it next month, in March, to upgrade to that one.”
CBP uses different versions of the NEC algorithm at different border crossings. The identification algorithm, which matches a photo against a gallery of images—also known as one-to-many matching—is used at airports and seaports. This algorithm was submitted to NIST and garnered the highest accuracy rating among the 189 algorithms tested.
NEC’s verification algorithm—or one-to-one matching—is used at land border crossings and has yet to be tested by NIST. The difference is important, as NIST found much higher rates of matching a person to the wrong image—or false-positives—in one-to-one verification compared to one-to-many identification algorithms.
One-to-one matching “false-positive differentials are much larger than those related to false-negative and exist across many of the algorithms tested. False positives might pose a security concern to the system owner, as they may allow access to imposters,” said Charles Romine, director of NIST’s Information Technology Laboratory. “Other findings are that false-positives are higher in women than in men, and are higher in the elderly and the young compared to middle-aged adults.”
NIST also found higher rates of false positives across non-Caucasian groups, including Asians, African-Americans, Native Americans, American Indians, Alaskan Indian and Pacific Islanders, Romine said.
“In the highest performing algorithms, we don’t see that to a statistical level of significance for one-to-many identification algorithms,” he said. “For the verification algorithms—one-to-one algorithms—we do see evidence of demographic effects for African-Americans, for Asians and others.”
Wagner told Congress that CBP’s internal tests have shown low error rates in the 2% to 3% range but that these were not identified as linked to race, ethnicity or gender.
“CBP’s operational data demonstrates that there is virtually no measurable differential performance in matching based on demographic factors,” a CBP spokesperson told Nextgov. “In instances when an individual cannot be matched by the facial comparison service, the individual simply presents their travel document for manual inspection by an airline representative or CBP officer, just as they would have done before.”
NIST will be assessing the error rates with regard to CBP’s program under an agreement between the two agencies, according to Wagner, who testified that a memorandum of understanding had been signed to begin testing CBP’s program as a whole, which includes NEC’s algorithm.
According to Wagner, the NIST partnership will include looking at several factors beyond the math, including “operational variables.”
“Some of the operational variables that impact error rates, such as gallery size, photo age, photo quality, number of photos for each subject in the gallery, camera quality, lighting, human behavior factors—all impact the accuracy of the algorithm,” he said.
CBP has tried to limit these variables as much as possible, Wagner said, particularly the things the agency can control, such as lighting and camera quality.
“NIST did not test the specific CBP operational construct to measure the additional impact these variables may have,” he said. “Which is why we’ve recently entered into an MOU with NIST to evaluate our specific data.”
Through the MOU, NIST plans to test CBP’s algorithms on a continuing basis going forward, Romine said.
“We’ve signed a recent MOU with CBP to undertake continued testing to make sure that we’re doing the very best that we can to provide the information that they need to make sound decisions,” he testified.
The partnership will also benefit NIST by offering access to more real-world data, Romine said.
“There’s strong interest in testing with data that is more representative,” he said.
Romine said systems developed in Asian countries had “no such differential in false-positives in one-to-one matching between Asian and Caucasian faces,” suggesting that data sets containing more Asian faces led to algorithms that could better detect and differentiate among that ethnic group.
“CBP believes that the December 2019 NIST report supports what we have seen in our biometric matching operations—that when a high-quality facial comparison algorithm is used with a high-performing camera, proper lighting, and image quality controls, face matching technology can be highly accurate,” the spokesperson said.
NIST and NEC did not immediately respond to questions Thursday.