NIST Releases Core Principles to Judge ‘Explainable AI’
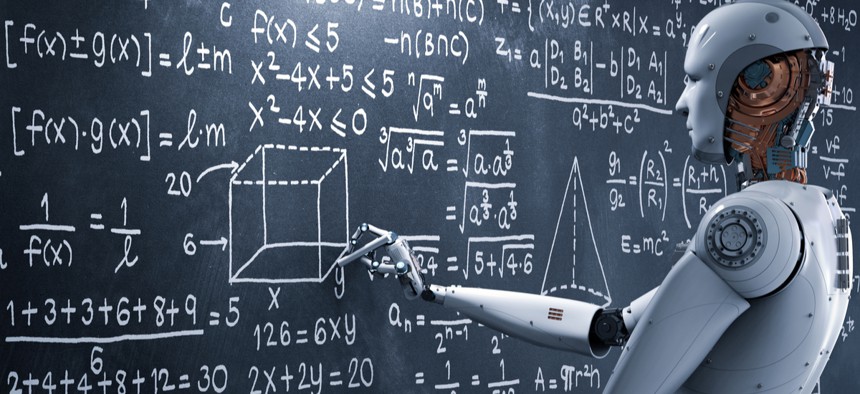
Phonlamai Photo/Shutterstock
The need for the technology to be trustworthy only grows as it’s increasingly adopted.
National Institute of Standards and Technology scientists carefully crafted and proposed four fundamental tenets for determining precisely how explainable decisions made by artificial intelligence are.
The draft publication released Tuesday—Four Principles of Explainable Artificial Intelligence—encompasses properties of explainable AI and is “intended to stimulate a conversation about what we should expect of our decision-making devices,” according to the agency. It’s also the latest slice of a much broader effort NIST is steering to promote the production of trustworthy AI systems.
“AI is becoming involved in high-stakes decisions, and no one wants machines to make them without an understanding of why,” NIST Electronic Engineer and draft co-author Jonathon Phillips said in a statement. “But an explanation that would satisfy an engineer might not work for someone with a different background. So, we want to refine the draft with a diversity of perspective and opinions.”
NIST’s four principles of explainable AI stress explanation, meaning, accuracy and what authors deem “knowledge limits.” As the agency states, they are:
- AI systems should deliver accompanying evidence or reasons for all their outputs.
- Systems should provide explanations that are meaningful or understandable to individual users.
- The explanation correctly reflects the system’s process for generating the output.
- The system only operates under conditions for which it was designed or when the system reaches a sufficient confidence in its output.
A final caveat included by the agency notes the last principle implies that “if a system has insufficient confidence in its decision, it should not supply a decision to the user.”
NIST’s draft also includes a call for patent claims “whose use would be required for compliance” with the guidance presented in the publication, as well as detailed sections on categories of explanation and an overview of explainable AI algorithms. Those bits largely outline core concepts of explainable AI to previous work conducted in the field—but the authors also devote a portion of the piece to compare explainability-driven expectations placed on the technology to those placed on people.
“[H]uman-produced explanations for their own judgments, decisions, and conclusions are largely unreliable,” authors wrote in the report, ultimately arguing that, “humans as a comparison group for explainable AI can inform the development of benchmark metrics for explainable AI systems; and lead to a better understanding of the dynamics of human-machine collaboration.”
Having previously conducted work demonstrating how human and AI collaboration can increase accuracy compared to either operating alone, Phillips further emphasized the point.
“As we make advances in explainable AI, we may find that certain parts of AI systems are better able to meet societal expectations and goals than humans are,” he said. “Understanding the explainability of both the AI system and the human opens the door to pursue implementations that incorporate the strengths of each.”
Phillips and the report’s other authors request that the public—including individuals with expertise in engineering, computer science, psychology, legal studies and beyond—provide comments and feedback on the draft by October 15.
“I don’t think we know yet what the right benchmarks are for explainability,” Phillips noted. “At the end of the day we’re not trying to answer all these questions. We’re trying to flesh out the field so that discussions can be fruitful.”