NIST Advocates Human-Centric Focus of AI Tech
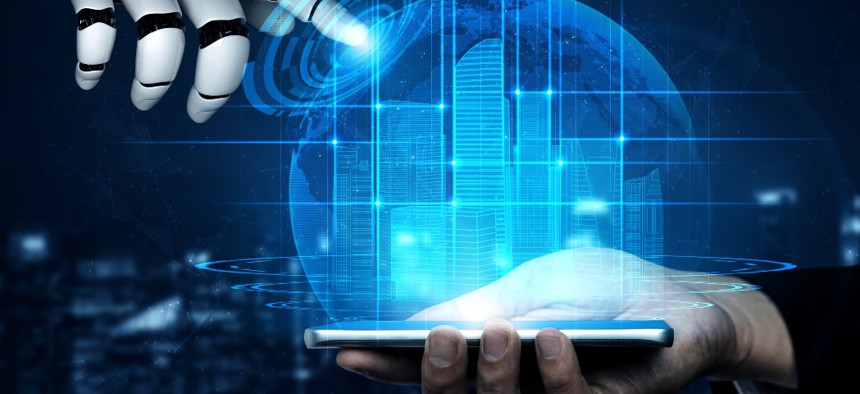
Pitinan Piyavatin / EyeEm/Getty Images
A new report delves into the biases that emerge in artificial intelligence, and best mitigation practices.
Federal agencies and officials utilizing artificial intelligence systems need to vigilantly monitor and control for systemic and racial biases included in machine learning technology, according to a new report from the National Institute of Standards and Technology.
This recommendation comes from an extensive report on how organizations and enterprises, both private and public, can cultivate better trust in artificial intelligence.
“Bias is neither new nor unique to AI and it is not possible to achieve zero risk of bias in an AI system,” the report begins. The document then categorizes biases in artificial intelligence in three groups: systemic, statistical and human. It then discusses mitigating each of these through testing, evaluation methods and other human factors.
“AI systems do not operate in isolation. They help people make decisions that directly affect other people’s lives,” said Reva Schwartz, the principal investigator for AI bias at NIST and one of the report’s authors. “If we are to develop trustworthy AI systems, we need to consider all the factors that can chip away at the public’s trust in AI. Many of these factors go beyond the technology itself to the impacts of the technology, and the comments we received from a wide range of people and organizations emphasized this point.”
The main approach NIST researchers advocate in the publication is called a “socio-technical” approach, which looks at machine learning as behavior patterns derived from datasets and algorithms but also feature human interaction.
“Organizations often default to overly technical solutions for AI bias issues,” Schwartz said. “But these approaches do not adequately capture the societal impact of AI systems. The expansion of AI into many aspects of public life requires extending our view to consider AI within the larger social system in which it operates.”
One socio-technical approach is to mitigate biases including system validation and prioritizing diversity among developers helping build machine learning algorithms.
“Numerous studies have touted the benefits of increased diversity, equity and inclusion in the workplace,” the report reads. “Yet, the AI field noticeably lacks diversity.”
Focusing on a human-centric design approach in artificial intelligence is also a recommendation, which emphasizes larger societal impacts of emerging technology.
“HCD [Human-centered design] works to create more usable products that meet the needs of its users,” the report reads. “This, in turn, reduces the risk that the resulting system will under-deliver, pose risks to users, result in user harms or fail.”