Los Alamos charts a new path on AI research with Venado launch
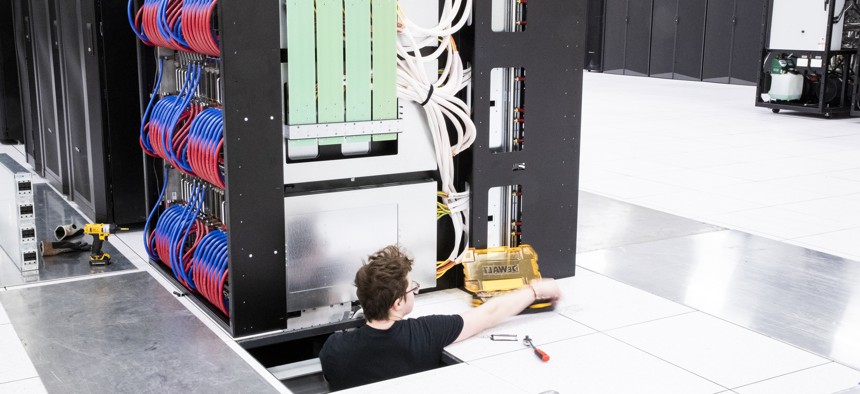
A technician works on the the installation of the Venado supercomputer at Los Alamos National Laboratory. Photo courtesy Los Alamos National Laboratory
Staff at the New Mexico laboratory took Nextgov/FCW inside their newest supercomputer installation and its potential to support artificial intelligence applications for both public and classified research.
LOS ALAMOS, N.M. — Researchers at the Los Alamos National Laboratory are poised to leverage new artificial intelligence technologies across scientific domains that impact national security research through the deployment of internal large language models and sophisticated data processors, a preview for the future of research in state-run laboratories.
In an exclusive interview with Nextgov/FCW, staff from Los Alamos discussed the laboratory’s future incorporating AI analyses into aspects of its scientific research process, from subjects like climate change to molecular dynamics.
“It's a joint investment from both the weapons side of the house, and the science and theory, computation side,” Galen Shipman, a computer scientist at Los Alamos National Laboratory, told Nextgov/FCW. “And so longer term, we would expect that this type of capability will be directly impacting both those missions.”
This initiative began with the April installation of a new supercomputer from NVIDIA and Hewlett-Packard Enterprises. The machine, Venado, is the latest hardware installation in LANL’s computing portfolio and marks a new chapter for the lab: introducing AI-powered computation into scientific modeling and simulation.
Central to the new system is NVIDIA’s proprietary Grace Hopper computer processing chips and associated hardware.
“The laboratory wanted to make a significant statement in that we are leading in computing as a national laboratory,” Jim Lujan, the HPC program manager at LANL told Nextgov/FCW. “We are already investing in significant capability with the Grace Hopper technology, and not just for our modeling and simulation capability, but now that could be directly applied for our AI or anticipated AI workflows.”
NVIDIA’s central processing and general processing units are at the heart of Los Alamos’s plans to incorporate AI and machine learning into their operations. Venado was procured precisely to support the NVIDIA chips’ computing capabilities, which in turn are designed to provide the memory bandwidth and computing speeds AI programs require.
“What Venado brings, and what some of the research that we've done in machine learning here at the laboratory brings to bear on this challenge, is that we can conduct very high fidelity interatomic potential simulations that take quite a bit of time,” Shipman said.
The interatomic potential Shipman refers to is the mathematical formulas that characterize the energy output when two or more atoms interact together. Simulating these interactions at a computational level is one way scientists can predict how molecules can interact under different circumstances.
Usually, Shipman said, the computational simulation of atoms is very expensive, and choosing to offset the cost stands to impact the accuracy of the results. AI-enabled processing can change that.
Data generated from these high-quality simulations that mimic realistic scenarios is then used to train machine learning models to draw confident conclusions at faster speeds than alternative approaches to experiment modeling.
“We get the speed of inference,” Shipman said. “And it's actually faster than what we would get in our traditional approach, which has much less fidelity and much higher error. And so this is actually bringing machine learning directly into the loop of our bread and butter, which is high performance simulation.”
Climate applications
Beyond molecular dynamics, AI-powered simulations can also help with the extensive meteorological and ocean modeling conducted at LANL. Bringing potential climate scenarios to life on a digital level also demands gargantuan amounts of data, which can take months without AI and machine learning.
“If you're worried about climate change, that is not all about but it's predominantly about the ocean, understanding the long term effects of Co2 emissions on the ocean,” Shipman said. “Those simulations are extremely expensive, particularly at high resolution. This becomes extremely important to understand climate impacts at very high resolution when you're starting to think about adaptation.”
Ultimately, the introduction of advanced AI capabilities drives both high performance simulations and precision data generation that helps breathe new life into the scientific theories and concepts being explored in a given experiment.
Plans for Venado and its AI computing capabilities expand into the lab’s other mission areas. LANL’s history is inextricably intertwined with national security needs. Famed as the birthplace of the atomic bomb that ultimately brought World War II to a close, LANL is now one of the three U.S. laboratories that works alongside the Department of Energy’s National Nuclear Security Administration to manage the nation’s stockpile of nuclear weapons maintained by the government.
Shipman and Lujan both said that eventually, Venado and the AI technology it supports will handle classified work.
“Part of the acquisition goals for Venado were, at some point during the life of Venado, we would transition a portion of the system to be able to facilitate even further research in the classified [areas],” Lujan said. “So we’ll cleave off a portion of Venado and transition it into the classified environment to support that mission.”
Classified missions
The key distinction between “classified” and “unclassified” research areas in the context of U.S. national laboratories boils down to the importance of a given project to national security missions. Unclassified research is typically published and available in peer-reviewed journals and can include a swath of topics like nanotechnology, climate science, fuel cell development and theoretical physics.
The National Nuclear Security Administration broadly characterizes research within any discipline as having the potential to be deemed “classified.” The key distinction is that classified research relates to individual laboratories’ national security missions.
Sometimes, however, these subjects overlap.
“You can study national security problems at an unclassified level too,” said Nick Generous, a LANL deputy group leader and scientist with a focus in biology. “Unclassified doesn't mean that it doesn't have national security relevance or importance.”
Research teams at LANL have already been employing a variety of applied machine learning softwares in their work, most recently seen with the July announcement of a partnership with OpenAI. Shipman said that Pytorch and Tensorflow, both open-source software libraries that help programmers create machine learning models, are regularly used in lab research, along with proprietary NVIDIA tools.
OpenAI brings more generative offerings to help with the fundamental programming of frontier models designed for specific research applications, focusing more on the company’s commercial and beta technology to understand the intersection of innovation and risk. For Generous, this would serve him in wrangling large sets of data for research in biosciences.
“[The] most immediate applications as a scientist [is] it can help me code things better, or maybe you can use it to help summarize papers or help even edit documents and things like that,” Generous said. “But it also got us thinking about what are some of the potential risks that might be associated with this technology.”
Following more experience and time using Venado to help train foundation models, Generous said the outcome will dictate whether AI technology will be suitable for use in more critical research. Unlike the Pytorch and TensorFlow examples, however, OpenAI will not run on Venado processors.
“That's kind of what this collaboration and partnership is meant to be about, is trying to understand how to evaluate their technology and use it in the lab and also then mitigate that risk,” he said.
This does not mean AI tools are ready to interact with the U.S. stockpile reserves. Given the obvious security risks posed by leveraging AI in any sensitive research arena, LANL scientists are taking a steady approach to deployment. Generous noted that a first step would be training a biological foundation model, and using the lessons learned to ensure machine learning systems are the correct solution –– especially if the software isn’t originally intended to work within certain fields.
“Before we can really go down all these pathways to address some of these national security implications, we really have to start looking at just that first step of implementing the infrastructure and then getting these models running, training them, learning how to use that and apply that to our domain spaces, because a lot of the domains that we're playing in aren't areas necessarily that companies will,” he said. “So while the technology might be mature in some areas, it's relatively new into the application term, mission spaces and domain areas.”
Shipman said that while Venado’s current focus is on using its processing power to leverage artificial intelligence in unclassified areas, the modeling and simulation capabilities offered by both the hardware and software will be “critical” for efforts in core materials science research. Materials science work at LANL is often informed by the needs of the stockpile stewardship mission central to the lab.
Keeping it safe
Guardrails for utilizing AI and machine learning systems in sensitive domains could range from omitting select datasets to implementing certain controls on inputs and outputs, according to Generous. Shipman added that the scarcity of historical data suitable to train frontier models often requires the use of responsibly generated and verified synthetic data to help a given AI model learn.
“A lot of times we don't have enough data. If you're talking about, in particular, experimental data, it can be few and far between,” Shipman said. “So we often rely on trusted simulation capabilities that have gone through a fairly rigorous validation [and] verification process to generate these datasets.”
One way LANL researchers confirm the AI models they are using are producing accurate results is to train multiple models on the same data simultaneously and compare the outputs. This method produces a helpful benchmark in addition to referencing historical data amassed by previous LANL work and works to mitigate errors lurking in deep neural networks.
“Unless we go to more traditional approaches, like Bayesian inference, where we have statistical tools and methods to understand errors, we don't have that for deep neural networks today,” Shipman said. “It's not quite as bad as a black box, but it's pretty close. So we have to fall back to tried and true techniques to understand the errors that are being introduced.”
Although the full potential of AI and machine learning technologies is still undetermined, LANL officials noted that AI softwares may eventually leverage Venado’s capabilities to explore classified research areas.
“Venado is clearly our large-scale focus right now for AI work in the unclassified, but obviously we are going to be looking at deploying AI in the classified outside of Venado,” Lujan said. “So it's entirely feasible that OpenAI may play in that role.”
These plans are far from set in stone. Improving the productivity of LANL applications developers to facilitate research efforts remains the starting goal for incorporating AI and machine learning systems. Shipman said that LANL will be partnering with multiple national laboratories to develop training data for a “copilot” AI capability meant to augment researchers’ work and prepare the lab for future researchers who have long used AI as an aid to their research.
“This could be transformative for our application developers, particularly those that we anticipate coming out of university over the next few years that will just be accustomed to using these tools already,” Shipman said. “We need to be ready at the laboratory and have these technologies available to our application developers.”
Weapons development
In addition to a copilot large language model function, LANL is also working on two projects that would train LLMs to work within the weapons science community. The first would manifest as a chatbot and aim to seek information of interest across an online document repository for the LANL weapons science researchers. The second would look to train LLMs on weapons-specific topic to provide a chatbot functionality for onboarding and training new scientists in the weapons research domain.
On the regulatory level, the NNSA and Department of Energy are still assessing how AI and automation can best fit into a national research setting.
“As with many novel technologies, teams at NNSA are working to understand how we can safely, responsibly and securely benefit from emerging artificial intelligence capabilities to help protect the nation and continually improve the scientific capabilities of our national laboratories,” an NNSA spokesperson told Nextgov/FCW.
For now, however, LANL has no timeline to introduce classified information into Venado. NVIDIA’s Hopper GPU architecture will arrive at the lab in October 2024 and leverage AI computing capabilities for classified research domains. This new system will be separate from Venado.
The NNSA also said that it references the Department of Energy’s current agencywide guidance on AI tools in deploying automated systems. Among the best practices listed for AI and machine learning system integration is to omit nonpublic –– or classified –– information as a prompt or input on a commercial or open generative AI system.
Congress has recently taken action to outfit the national lab network with a stronger AI infrastructure. Sens. Joe Manchin, I-W.V., and Lisa Murkowski, R-Ark., introduced legislation on Monday that aims to allocate more funding to install multidisciplinary AI centers in select national labs, while also monitoring the risks associated with deploying AI systems in sensitive arenas, including the generation of nuclear, biological and chemical weapons.
Ultimately, Generous is excited about the prospect of further integrating AI in laboratories to expedite experiment development and evaluate results.
“It has the potential to be very transformative in advancing scientific research and in multiple different ways,” Generous said. “I think it's going to accelerate innovation.”
NVIDIA declined to comment on this story.