DHS's Biometric Rally Reveals Strong Capabilities Reliant on Camera Configuration
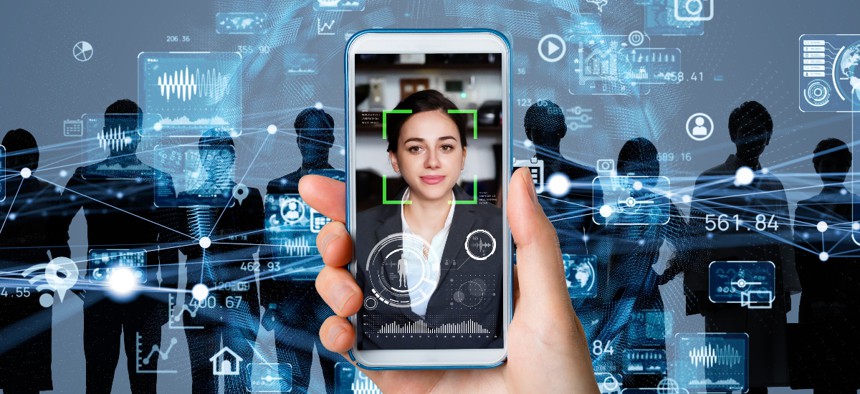
metamorworks/Getty Images
Researchers at the Department of Homeland Security’s Science and Technology Directorate explained how camera systems’ capacity for varied skin tones can make or break an accurate biometric reading.
The Department of Homeland Security’s Science and Technology Directorate is placing a heavy emphasis on accuracy in the facial recognition algorithms powering biometric devices used at security checkpoints in U.S. transit hubs.
The agency debuted research results from the S&T’s 2022 Biometric Technology Rally—held over 11 days at a test facility in Upper Marlboro, Maryland—evaluating the accuracy of facial recognition algorithms and technologies that were developed in collaboration with participating vendors.
The goals of this rally were to mitigate errors in the technology powering facial recognition devices, specifically when registering the correct human face amid a crowd of people and establishing wide-ranging biometric industry standards.
“With the rallies in particular, what we focus on is putting out a use case to industry describing an overall set of problems,” said Arun Vemury, the Biometric and Identity Technology Center lead at DHS S&T. “Defining measures and metrics that are relevant for end users and stakeholders, not just arbitrary error rates, like false positive identification rates and false negative identification rates.”
The agency chose 16 biometric systems to participate in S&T’s test. A key component of the 2022 challenge was to train these systems to accurately select an individual face in groups of people to mimic crowded public transportation stations. A system’s ability to do this would both enhance accuracy of the face being read as well as protect the privacy of external bystanders.
“We're basically looking for technology that could gather a single high quality picture for each person in the group,” Yevgeniy Sirotin, technical director of the Identity and Data Sciences Lab at S&T’s Maryland Test Facility, said to reporters at a Thursday briefing.
Sirotin explained that processing time, group size and user satisfaction were among the performance metrics used to evaluate a system. Most of the biometric systems succeeded in only processing a specific, consenting participant under a three second benchmark.
“A lot of the systems successfully refrained from sending us any images or collecting any images for people outside of the capture zone, which speaks to the fact that, even though you can have perhaps a lot of biometric systems deployed within a particular environment like an airport terminal, they can be configured—when that's a requirement—to capture images only from people that are actually actively using [the system],” Sirotin said.
A more unique feature in these biometric systems was the incorporation of skin tone into the algorithms. Researchers aimed to take a calibrated reading of an individual’s skin color based on technology initially used in dermatological environments.
Accuracy readings for skin tone faced more hiccups than other biometric data. Sirion noted that, for individuals with relatively lighter and darker skin tones, the algorithms suffer occasional errors. Medium skin tones performed the best for accuracy, which he attributed to a need for specialty cameras designed to accurately capture an individual’s coloring.
“The net reflectiveness of your skin determines how many photons get reflected back into the camera, and fundamentally this is a physics problem,” he said.
That differing reflection of photons can result in some skin tones becoming overexposed in images, while others are underexposed. Sirion noted that such differentiation demonstrates the need for biometric cameras to be properly configured.
Vemury said that industry entities understand the need for suitable cameras to capture an image that best suits the specific biometric algorithm in use at a given location, and said that there has been “substantial improvement” in camera systems over the years of researching biometric technologies.
“Part of this whole effort [of] why we do this was, if you look at the NIST evaluations, you don't realize that cameras are a problem,” Vemury said. “Through these types of evaluations that we do here, you get a better understanding of where the app errors happen in the overall system. And we have seen industry respond and provide better and better camera systems.”
Despite the broadly positive results for all biometric algorithmic systems, not all technologies are ready for deployment at local airports or entry ports. The S&T published the results of the rally publicly online, and is open to acting as a broker between the companies manufacturing the biometric systems and interested government parties.
“The intention is to put out objective, clear information to the public, to people, so that they can make better decisions in the acquisition process,” Varun said. He also noted that the transition for a controlled lab to real-world settings is bumpy, and that more errors––particularly human error––are likely to occur.
“Putting in the infrastructure, acquiring the systems, deploying them, making sure you have processes and procedures for people in the field, to be able to understand how to use it or how to resolve errors…there's a lot of additional work that comes into play there,” Varun said.