Fake Data Sets Could Safeguard Privacy
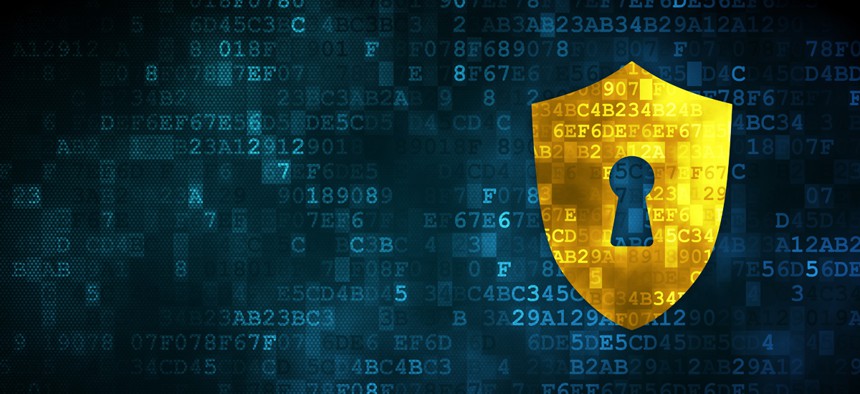
Maksim Kabakou/Shutterstock.com
Synthetic datasets allow researchers to study social systems without compromising individual identities—but how reliable is the information they’re using?
The first U.S. Census was carried out in 1790 to count the population in each state and assign seats in Congress accordingly. Since then, the Census Bureau has expanded its mission, collecting information about occupation, education, income, geographical location, and other personal data, which researchers use to inform economic and social policies. As more detailed information is collected, the datasets become more useful—but confidentiality becomes harder to preserve.
“The Census Bureau has a lot of data that is potentially very valuable, and the more use we make of it, the more value it can be to society,” said Margaret C. Levenstein, executive director of the Michigan Census Research Data Center. “So that is a tradeoff—how do you make the most use of it but maintain the confidentiality?”
A research team led by Jerry Reiter, a professor of statistics at Duke University, and John Abowd, a professor of economics at Cornell University, has developed an innovative approach to solve this problem using not real, but synthetic data: simulated data generated from statistical models.
There are basically two ways to reduce the risk of a confidentiality breach, Abowd explained. The familiar approach is to perform an analysis on confidential data and then add random error to the output of the analysis. Introducing random error in the output is necessary to reduce the chance that information about any individual will be revealed. But sometimes the random error precisely masks the features that researchers are interested in. Another way, that gets around this problem, is to implement privacy protections on the input of an analysis, by modifying the dataset itself. Reiter and Abowd chose the latter approach.
In their approach, the researchers feed the original Census data, which is kept confidential, into a complex statistical model that generates a simulated population that has the same general features as the original data. If you have a confidential dataset of 100 individuals' ages and incomes, for example, a corresponding synthetic dataset composed of 100 imaginary individuals would have the same mean age and mean income as the original. One of the major challenges is to create synthetic data that is statistically identical, but not an exact replica, of the original data.
“Any query that can be asked of the confidential data can also be asked of the synthetic data,” Abowd said. Because the synthetic data represent imaginary individuals, there is low risk in making synthetic data public.
The synthetic data are often used to develop and test computer code for analyses. But ultimately, any analysis on synthetic data needs to be verified on the original dataset. So, the researchers developed a "verification server"—an intermediary computer—to perform the same analysis on the original confidential data. The verification step determines whether the results of the analysis on synthetic data are also true for the original data. “The validation is a way of making sure that the assumptions that were built into the synthetic data are not driving the results, as opposed to the thing that the person is trying to study,” said Levenstein.
The Synthetic Longitudinal Business Database (SynLBD), released in 2011, is the result of their work, and the first ever record-level database on business establishments released by the Census Bureau. The Census Bureau collects information about businesses—the value of their output, how many employees they have, how much they spend on research and development, and so on. For businesses, privacy is important mainly because of strategic concerns. They might not want their competitors, customers, or suppliers to know exactly what is going on with their business, Levenstein explained.
The identity of businesses is hard to disguise by simply adding noise to a dataset. “Businesses are very different from one another,” Levenstein said. “You cannot hide General Motors or Walmart in a dataset. It’s too hard to anonymize the data in a way that would still make them useful. If you did enough masking, you’d be masking what’s important about employment and economic output in America. So you can’t do that.” Instead, the research team created the SynLBD, a database of synthetic data about businesses, which allows researchers to develop a better understanding of entrepreneurship, and to study the dynamics of the American economy—and what is causing it to grow or not—without revealing confidential information about individual businesses.
The team also created a database called the Survey of Income and Program Participation (SIPP) Synthetic Beta Data Product, which allows researchers to do important analyses about food security, poverty, income inequality, and other issues, Levenstein explained. The (nonsynthetic) Survey of Income and Program Participation has been going on for about 40 years, she said. “If you have that kind of information over a long period of time for a person, it increases the probability that the person could be re-identified. So we have created a synthetic version of SIPP.” The synthetic database allows any researcher in the community to study important questions that can have implications for government programs such as food stamps. Without the synthetic data, much of this research would be logistically difficult or impossible. “The realistic alternative to publishing the SIPP synthetic data is suppression (no publication of any form of the linked administrative data) with individual researchers proposing projects on a one-by-one basis for access to the confidential data,” said Abowd. “Those projects would have to be approved by the Census, Internal Revenue Service and Social Security Administration.”
Although examples of synthetic data applications exist, other researchers aren’t yet sold on that approach to privacy. Yves-Alexandre de Montjoye, a graduate student at MIT who works on data from mobile phones, agreed that synthetic data are useful for testing algorithms, but pointed out that the method can never preserve all the interesting relationships and properties of the original data. Gerome Miklau, an associate professor of computer science at University of Massachusetts at Amherst, made a similar point: “The tension is that you can’t create synthetic data that’s accurate for everything.”
Reiter recognizes the problem. “Ideally, the statistical models that are used to generate the synthetic data would preserve the properties or relationships of interest.” But even if they don’t, he added, the researcher’s time isn’t wasted. If the results of the analyses did not match, a user could apply for access to use the restricted data, but only in a secure computing environment.
Creating a model that generates useful synthetic data is not a trivial task, de Montjoye pointed out. And the appropriate models are likely to be specific to the question that an investigator is asking, so multiple models—and synthetic datasets—may be needed. In other words, a one-size-fits-all synthetic dataset may be simply too good to be true. To address this challenge, the team has been improving the synthetic databases iteratively. Abowd explained that every version is improved based on the results of user validation tests. For example, when a researcher validates analyses on synthetic data against the confidential data, the validation test might indicate that the two results do not match; in that case, a new synthetic dataset could be generated that better preserves the specific features of interest in the confidential data. “The models that users submit for validation are used to improve the next release of the synthetic data,” Abowd said. SIPP is in its sixth iteration and SynLBD is in its second iteration.
The synthetic data approach has several advantages over the method of adding random error to the output of an analysis, Reiter pointed out. First, for the query systems to satisfy the privacy definition called “differential privacy,” there must be a limit on the number of queries that can be made on the original dataset—the greater the number of queries, the greater the risk of a confidentiality breach. In other words, the query systems approach comes with a finite “privacy budget,” which becomes exhausted as more and more queries are made. Once the privacy budget is exhausted, the dataset can no longer be used. In contrast, there is no limit to the number of analyses or queries that can be made on a synthetic dataset.
Another advantage is that, unlike the query systems approach where the researcher only sees aggregate results, the synthetic data approach allows the researcher to see a full dataset. Social scientists often want data on an individual household or an individual business, Levenstein said. “That is really useful for understanding the impact of policies and changes in the economic and social environment on behavior, which is often hard to tease out if you are looking at aggregates.” Reiter is a staunch advocate of sharing individual-level data. “Individual-level data have enormous potential benefits," he said. It’s difficult to know what analysis you really want to do unless you have some raw data in front of you,” he explained.
“The main thing is that synthetic databases increase the value of Census Bureau data products while still protecting their confidentiality,” Levenstein said.
Indeed, the value of datasets increase the more they are used, and the primary purpose of statistical agencies is to collect and curate data as a public good: “One person’s using them doesn’t diminish their value to other people, and so we’d like, when we invest a lot of resources in creating this data, for that to be available to the research community for general scientific advance,” Levenstein said. “If the Census data were completely confidential, there would be no point to it,” Abowd said.
The key paradox is how to keep individual information private and make it accessible at the same time, and, as Miklau put it, “the extent to which that’s possible is still not known.”
(Image via Maksim Kabakou/ Shutterstock.com)