Computers Have Found a Better Way to Spot Emailed Malware, Researchers Say
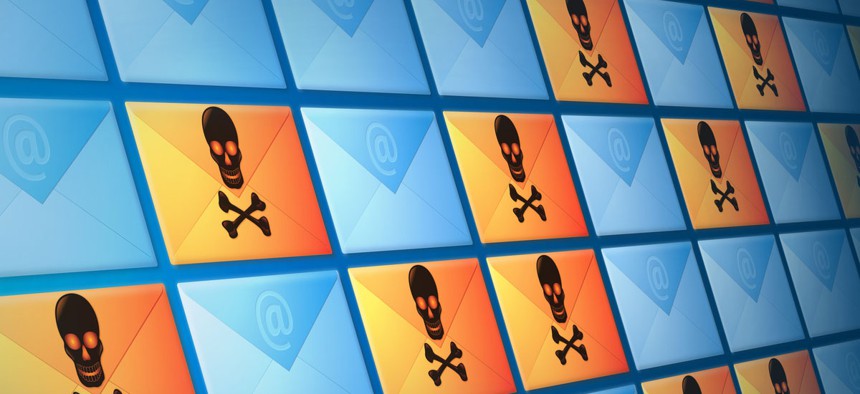
photoinnovation/Shutterstock.com
Somehow no one thought of applying machine learning to malicious email in exactly this way. But the results are big.
Look out, McAfee; the next big cybersecurity software could be coming out of Israel. A group of researchers from Ben-Gurion University has published a new method for detecting malicious emails that they say outperforms 60 top-selling anti-virus programs.
Most anti-virus engines examine specific parts of email, such as attached files, as they look for malicious code that could disrupt a user’s computer if it were executed. It’s kind of like checking someone’s carry-on for contraband. While that’s the most logical place for a border guard to look, it’s hardly the only place a smuggler might hide something. Current anti-virus software misses key areas in email that are increasingly likely to carry bad code.
“Existing email analysis solutions only analyze specific email elements using rule-based methods, and don’t analyze other important parts,” Nir Nissim, head of the David and Janet Polak Family Malware Lab at Cyber@BGU, said in a press release. For instance, the number and size of attachments is a typical giveaway of a suspicious email, as is the number of recipients, since most email attackers are seeking the largest number of potential victims. But those aren’t the only indicators.
Led by Aviad Cohen, a Ph.D. student and researcher at the BGUMalware Lab, the researchers took 33,142 emails, about one-third of which were malicious, and applied various machine-learning methodologies to find common indicators of bad email that popular virus-detecting software packages such as Kaspersky, McAfee, and BitDefender missed. They dubbed the resulting tool Email-Sec-360°.
“The results show that malicious emails can be detected effectively when using our novel features with machine learning algorithms. Moreover, our novel features enhance the detection of malicious emails when used in conjunction with features suggested by related work,” the researchers write in their paper.
For instance, some HTML tags in the body of an email, such as “<iframe>,” a tag that opens a window into another webpage but without linking to it, is one that most filters ignore. Another underlooked indicator are links where the text in the URL is different from link it goes to, also attachments where the extension type, such as .PDF or .XLSX, doesn’t match the actual type of file.
They identify some 100 top features that could enable machine-learning algorithms to pick up malware much better. They’re the sorts of things that you wouldn’t know to look for if you couldn’t take a scan of a ton of malicious emails for some common feature, a feature beyond just malicious code in and of itself.
“In future work, we are extending our research and integrating analysis of attachments such as PDFs and Microsoft Office documents within Email-Sec-360°, since these are often used by hackers to get users to open and propagate viruses and malware,” said Nassim.