Models, models everywhere
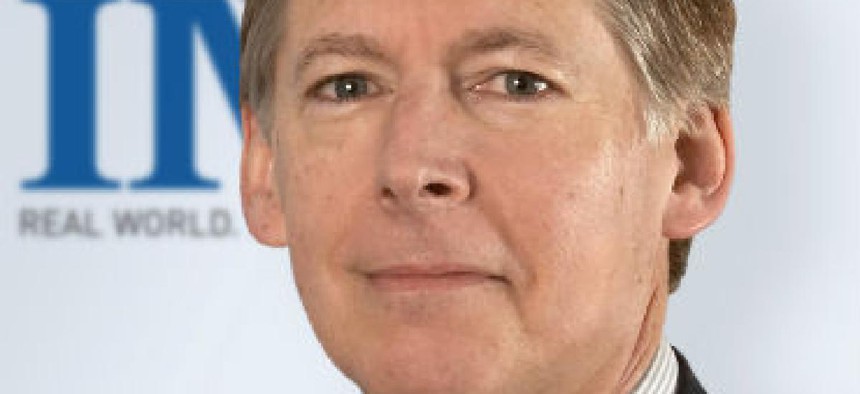
This excerpt from "Left Brain, Right Stuff" explains the value and limitations of decision models.

The insight that even simple models can lead to surprisingly accurate decisions has been around for some time. In 1954, Paul Meehl, a psychologist at the University of Minnesota, compared expert forecasts with the predictions of simple statistical models. Although the models used only a fraction of the data available to the experts, they were almost always more accurate. A number of similar studies have reached the same conclusion. Even seemingly crude models often do very well.
Models are accurate in part because they avoid common errors that plague humans. People suffer from the recency bias, placing too much weight on recent information while downplaying earlier data. They pay too much attention to information that is readily available. They're also unreliable: Give someone the same information on two different occasions, and he or she may reach two rather different decisions. Models have none of these problems. They can also crunch copious amounts of data accurately and reliably.
For decades decision models have made important contributions to a wide variety of fields. Colleges rely on models to evaluate applications for admission. By using formulas that assign weights to variables -- high school grade point average, test scores, recommendations and extracurricular activities -- colleges can make better predictions of academic success than by relying on a one-at-a-time review of each candidate.
Banks use models to grant loans. In bygone times, bankers relied on the three Cs: credit, capacity and character. They asked: Does the applicant have a strong credit record? Does his monthly income leave enough money, after other expenses, to make the payments? Does she seem trustworthy? Those aren't bad rules of thumb, but bankers, like everyone else, are prone to error. Models do a better job of predicting whether a loan will be repaid, and by updating them continually with the latest information, we can make them even more accurate over time.
In recent years the use of decision models has surged. The combination of vast amounts of data -- stored in places like the NSA's Utah Data Center [a facility featured early in Rosenzweig's book] -- and increasingly sophisticated algorithms has led to advances in many fields. Some applications are deadly serious. Palantir, based in Palo Alto, Calif., analyzes masses of financial transactions on an ongoing basis to detect money laundering and fraudulent credit card usage. It also serves the U.S. military by examining photographic images on a real-time basis to spot suspicious objects that might be roadside bombs.
San Francisco-based Climate Corp. gathers years of data about temperature and rainfall across the country to run weather simulations and help farmers decide what to plant and when. Better risk management and improved crop yields are the result.
Other applications border on the humorous. Garth Sundem and John Tierney devised a model to shed light on what they described, tongue firmly in cheek, as one of the world's great unsolved mysteries: How long will a celebrity marriage last? By gathering all sorts of facts and feeding them into a computer, they came up with the Sundem/Tierney Unified Celebrity Theory. With only a handful of variables, the model did a very good job of predicting the fate of celebrity marriages over the next few years.
Models have shown remarkable power in fields that are usually considered the domain of experts. Two political scientists, Andrew Martin and Kevin Quinn, developed a model to explain recent Supreme Court decisions -- whether the nine justices would uphold or overturn a lower court ruling -- based on just six variables. To see whether the model could actually predict decisions, University of Pennsylvania law professor Ted Ruger applied it to the upcoming Supreme Court term. Separately, he asked a panel of 83 legal experts for their predictions about the same cases. At the end of the year, he compared the two sets of predictions and found that the model was correct 75 percent of the time, compared to 59 percent for the experts. It wasn't even close.
Models can even work well for seemingly subjective tasks. Which would you think does a better job of predicting the quality of wine: a connoisseur with a discerning palate and years of experience, or a statistical model that can neither taste nor smell? Most of us would put our faith in the connoisseur, but the facts tell a different story. Using data from France's premier wine-producing region, Bordeaux, Princeton economist Orley Ashefelter devised a model that predicted the quality of a vintage based on just three variables: winter rainfall, harvest rainfall and average growing season temperature. To the surprise of many and embarrassment of a few, the model outperformed the experts -- and by a good margin.
These last two examples were described by Yale law professor Ian Ayres in "Super Crunchers: Why Thinking-by-Numbers Is the New Way to Be Smart." Ayres explained that models do so well because they avoid common biases. Not surprisingly, he mentioned overconfidence, noting that people are "damnably overconfident about our predictions and slow to change them in the face of new evidence." Decision models, of course, don't suffer from such biases. They weigh all data objectively and evenly. No wonder they do better than humans.
So are decision models really "the new way to be smart"? Absolutely. At least for some kinds of decisions.
But look back over our examples. In every case, the goal was to make a prediction about something that could not be directly influenced. A model can estimate whether a loan will be repaid but can't change the likelihood that a given loan will be repaid on time. It won't give the borrower any greater capacity to pay or make sure he doesn't squander his money the week before payment is due. A model can predict the rainfall and days of sunshine on a given farm in central Iowa but can't change the weather. A model can estimate the quality of a wine vintage but won't make the wine any better. It can't reduce the acidity, improve the balance, or add a hint of vanilla or a note of cassis.
For the sorts of situations in which our aim is to make an accurate estimate of something we cannot influence, models can be enormously powerful. But when we can influence, the story changes. Our task isn't to predict what will happen but to make it happen.