How Big Data Can be Used to Fight Unfair Hiring Practices
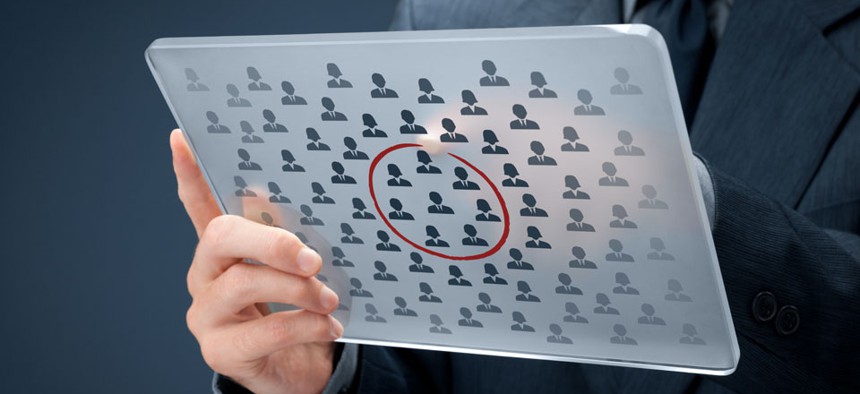
Jirsak/Shutterstock.com
Big data may be fraught with peril, but its evangelists say its use could help remedy common—and often unseen—discriminatory behavior. But only if employers really want that.
Humans are fallible, biased creatures, and even the most well-intentioned hiring managers have a strong tendency to hire "look like me, act like me" candidates.
Those unintended prejudices in recruitment—whether racial, gendered, or economic—are shortcomings that a growing number of big-data firms are hoping they can help solve with their massive number-crunching operations. By mining troves of personal and professional data, these companies claim they can not only match employers with A-plus job candidates, but help close diversity gaps in the workforce, too.
"Big data in the workplace poses some new risks, but it may yet turn out to be good news for traditionally disadvantaged job applicants," said David Robinson, a principal at Robinson + Yu, a consulting group that works to connect social justice and technology.
Still, concerns abound. Earlier this year, the White House released a landmark report on big data, warning that the exploding enterprise could—intentionally or not—allow companies to use data to discriminate against certain groups of people, particularly minorities and low-income groups. That's also the fear of the Federal Trade Commission, which held a workshop last week exploring the concept of "discrimination by algorithm."
"Big data can have consequences," FTC Chairwoman Edith Ramirez said. "Those consequences can be either enormously beneficial to individuals and society, or deeply detrimental."'
But firms working to leverage big data for HR departments strike a more optimistic tone.
"We think that there's a huge upside to it," said Kyle Paice, vice president of marketing for Entelo, a San Francisco start-up that uses predictive analytics to connect businesses with desired talent likely looking for a new job.
Paice said Entelo's experience is that companies generally want to be more inclusive, as studies show such workforces are more innovative, more profitable, and have less turnover. But those companies often have difficulty fielding diverse applicant pools, he said, such as female candidates for computer-developer positions (women in science careers are commonly subject to negative bias). So Entelo serves as a sort of middle man, using its data to cull from a wider net of talent and surface a broad array of applicants who otherwise might not even know about an open position.
But data isn't as virtuous as some of its boosters might like it to be. Just as hiring managers are often unaware of their biases, data can also have unintended, adverse blind spots, said Solon Barocas, who is conducting postdoctoral research on the ethics of machine learning at Princeton's Center for Information Technology Policy and just coauthored a study on big data's "disparate impact."
"Data mining works by setting up specific examples from patterns in the past that instruct the future," Barocas said. "The way you assemble those examples will determine the models you have and the errors you make."
That formula can easily perpetuate undetected bias, he added.
"If you're a company that doesn't have a history of hiring women or minorities, your model will tell you that these people are not especially well qualified. Even if you're simply trying to minimize turnover, what you might do is systematically exclude certain groups that are poor or of a certain ethnicity."
Big-data firms contend that those scenarios are unlikely, and often actively avoided. Consider the story of Evolv, another San Francisco start-up in the business of helping its clients hire the right people. The seven-year-old company also deploys data to help employers improve their management practices.
Evolv, like Entelo, believes its data can dispel the biases of things like gut feeling to provide better assessments for industries looking for talented, diverse hires. But the company says it strives to avoid replicating similar, data-based prejudices by tweaking its "scoring algorithm" to ensure it doesn't unintentionally discriminate against certain groups.
For example, Evolv's data indicates that workers who live farther away from the office are more likely to quit. But that data is discarded from its "secret sauce" because it could have an adverse impact of ignoring certain socioeconomic communities, which are often geographically stratified, said Michael Housman, the company's chief analytics officer.
"Distance [from work] is something we don't use in our algorithm because it's a can of worms," Housman said. "We don't want to go close to anything that could discriminate against the poor or others in disadvantaged areas."
Part of that reticence stems from a fear of breaking federal equal-opportunity laws, as using any data set that blocks out protected groups is problematic. But the company also abides by a "big-data code of ethics" that strives to include all groups in its data sweeps, Housman said.
Barocas, however, contends that whatever the intentions, big data still suffers from technical pitfalls that can lend itself to bias, no matter the good intention of the employer. Overcoming that requires sizable investments in getting the data not just right but granular and contextualized, as well as an active commitment to diversity as an end goal in of itself—and not just for the purpose of finding the best person for any given role.
Done right, big data "can be an enormous force for good," Barocas said. "We don't want to discourage people from doing that good work. We want to make sure there's no assumption that by just using data, you're suddenly fair."
At the very least, big data is better than most current practices, said Daniel Castro, a senior analyst with the Information Technology and Innovation Foundation who participated in the FTC's big-data panel last week.
"All data is biased. All data is interpreted. It's shaped by human factors," Castro added. "It's not that we don't have biases in the data, it's that we have fewer biases and ways to mitigate those biases to get us to a better place than we are today."
(Image via Jirsak/Shutterstock.com)