Data Scientists Keep Forgetting the One Rule Every Researcher Should Know by Heart
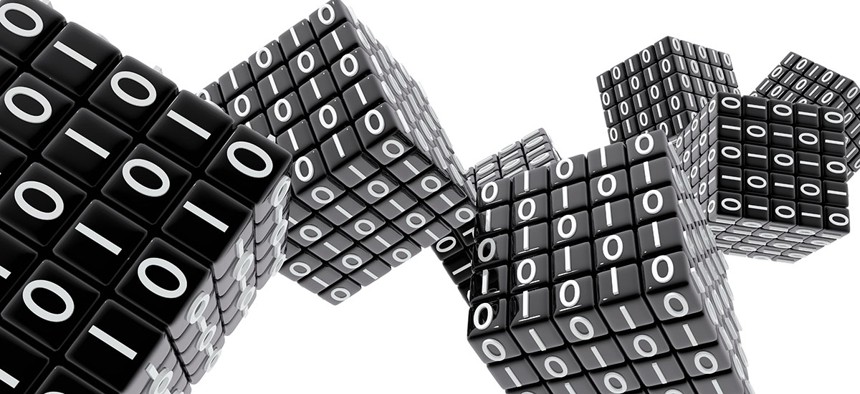
Genialbaron/Shutterstock.com
Correlation does not imply causation.
The results are in: Skipping breakfast is associated with heart disease. But that doesn’t necessarily mean breakfast deserves its reputation as the most important meal of the day.
Harvard University medical researchers have concluded that American men between the ages of 45 to 82 who skip breakfast showed a 27% higher risk of coronary heart disease over a 16-year period. However, rather than directly affecting health, eating breakfast may more simply be a proxy for lifestyle.
People who skip breakfast tend to lead more stressful lives. Participants in the study who skipped breakfast “were more likely to be smokers, to work full time, to be unmarried, to be less physically active, and to drink more alcohol,” Harvard researchers report. In other words, the link between breakfast and health may not be causal.
This is a perfect example of why a certain scientific mantra is often repeated: Correlation does not imply causation. Yet data scientists often confuse the two, succumbing to the temptation to over-interpret. And that can lead us to make some really bad decisions—which could put a damper on the enormous value of making predictions from data.
Predictive analytics draw on data to predict likely outcomes—whether a criminal is likely to be a repeat offender, say, or the chances of recovery for medical patients. The practice has major implications for improving healthcare, financial services, law enforcement, government, and manufacturing, among other industries.
Yet there’s a real risk that advances in predictive analytics will be hampered by our overly interpretive minds. Stein Kretsinger, founding executive of Advertising.com, offers a classic example. In the early 1990s, as a graduate student, Stein was leading a medical research meeting, assessing the factors that determine how long it takes to wean a person off a respirator. This was before the advent of PowerPoint, so Stein displayed the factors, one at a time, on overhead transparencies. The team of healthcare experts nodded their heads, offering one explanation after another for the relationships shown in the data.
But after going through several transparencies, Stein realized that he’d been placing them with the wrong side up—thus displaying mirror images of his graphs that depicted the opposite of the true relationships between data points. After he flipped them to the correct side, the experts seemed just as comfortable as before, offering new explanations for what was now the very opposite effect of each factor.
In other words, our thinking is malleable. People can readily find underlying theories to explain just about anything.
Take the incident of a published medical study that discovered that women who happened to receive hormone replacement therapy showed a lower incidence of coronary heart disease. Could it be that a new treatment for this disease had been discovered?
Apparently not. A subsequent experiment with proper controls disproved this conclusion. Instead, the current thinking is that more affluent women had access to the hormone replacement therapy, and these same women had better health habits overall. This sort of follow-up analysis is critical.
Businesses can also mistake effect for cause. For example, imagine an online car dealership that discovers website visitors who use a price calculator are more likely to eventually purchase a vehicle. This insight helps inform their predictions—it might be wise to offer a discount to customers who didn’t use the price calculator, for example, to increase the likelihood that they’ll buy a car. But it does not necessarily explain what factors influence customers’ decisions. It may be that eager, engaged consumers are naturally more inclined to explore the website’s features in general. So working to actively promote the price calculator wouldn’t necessarily help increase sales and could be a wasted effort.
Uber offers another useful example. The company discovered that, in San Francisco, more passengers request rides from areas with higher rates of prostitution, alcohol use, theft, and burglary. However, the company knows that crime itself is not necessarily causing this higher demand, even indirectly. Rather, their original hypothesis, even before the analysis, was that “crime should be a proxy for nonresidential population.” Higher-crime areas tend to have more people who don’t live in the city, who in turn need rides.
Prematurely jumping to conclusions about causality is bad science that leads to misinformed decisions–and the consequences could be a lot more worrisome than an unnecessary bowl of cereal in the morning. Luckily, avoiding this mistake is simple. Companies, researchers and governments can certainly use predictive analytics to drive some decisions—such as flagging patients who skip breakfast, so that healthcare providers can consider additional diagnostic or preventative measures. But we must avoid giving our gut instincts too much credit, and understand that our conjectures about the root cause of a predictive discovery require further analysis.
(Image via Genialbaron/Shutterstock.com)