The Dark Side of Big Data
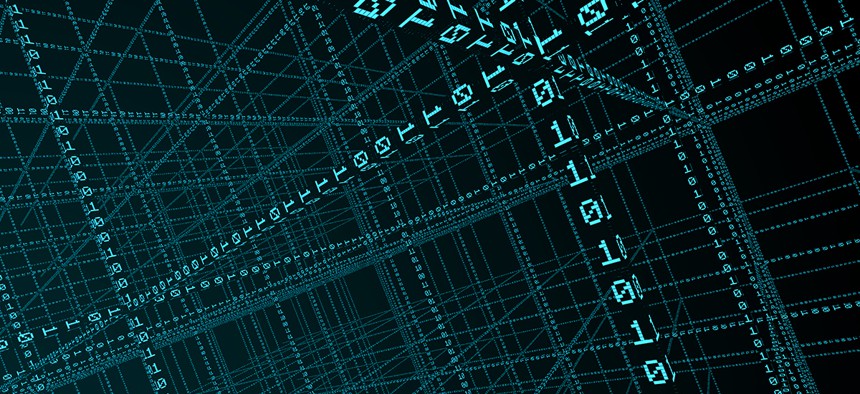
Mopic/Shutterstock.com
Surveillance and public-benefits programs gather large amounts of information on low-income people, feeding opaque algorithms that can trap them in poverty.
Big data can help solve problems that are too big for one person to wrap their head around. It’s helped businesses cut costs, cities plan new developments, intelligence agencies discover connections between terrorists, health officials predict outbreaks, and police forces get ahead of crime. Decision-makers are increasingly told to “listen to the data,” and make choices informed by the outputs of complex algorithms.
But when the data is about humans—especially those who lack a strong voice—those algorithms can become oppressive rather than liberating. For many poor people in the U.S., the data that’s gathered about them at every turn can obstruct attempts to escape poverty.
Low-income communities are among the most surveilled communities in America. And it’s not just the police that are watching, says Michele Gilman, a law professor at the University of Baltimore and a former civil-rights attorney at the Justice Department.
Public-benefits programs, child-welfare systems and monitoring programs for domestic-abuse offenders all gather large amounts of data on their users, who are disproportionately poor.
In certain places, in order to qualify for public benefits like food stamps, applicants have to undergo fingerprinting and drug testing. Once people start receiving the benefits, officials regularly monitor them to see how they spend the money, and sometimes check in on them in their homes.
Data gathered from those sources can end up feeding back into police systems, leading to a cycle of surveillance.
“It becomes part of these big-data information flows that most people aren’t aware they’re captured in, but that can have really concrete impacts on opportunities,” Gilman says.
Once an arrest crops up on a person’s record, for example, it becomes much more difficult for that person to find a job, secure a loan, or rent a home.
And that’s not necessarily because loan officers or hiring managers pass over applicants with arrest records—computer systems that whittle down tall stacks of resumes or loan applications will often weed some out based on run-ins with the police.
When big-data systems make predictions that cut people off from meaningful opportunities like these, they can violate the legal principle of presumed innocence, according to Ian Kerr, a professor and researcher of ethics, law, and technology at the University of Ottawa.
Outside the court system, “innocent until proven guilty” is upheld by people’s due-process rights, Kerr says: “A right to be heard, a right to participate in one’s hearing, a right to know what information is collected about me, and a right to challenge that information.”
But when opaque data-driven decision-making takes over—what Kerr calls “algorithmic justice”—some of those rights begin to erode.
As a part of her teaching, Gilman runs clinics with her students to help people erase harmful arrest records from their files. She told me about one client she worked with, a homeless African-American male who had been arrested 14 times.
His arrests, she said, were “typical of someone who doesn’t have a permanent home”—loitering, for example—and none led to convictions. She helped him file the relevant paperwork and got the arrests expunged.
But getting arrests off a person’s record doesn’t always make a difference. When arrests are successfully expunged, they disappear from the relevant state’s publicly searchable records database.
But errors and old information can persist in other databases even when officially corrected. If an arrest record has already been shared with a private data broker, for example, the broker probably won’t get notified once the record is changed.
In cases like these, states are nominally following fair-information principles. They’re allowing people to see information gathered about them, and to correct mistakes or update records.
But if the data lives on after an update, Kerr said, and “there’s no way of having any input or oversight of its actual subsequent use—it’s almost as though you didn’t do it.”
The pitfalls of big data have caught the eye of the Federal Trade Commission, which hosted a workshop on the topic in September. Participants discussed how big-data analysis can include or exclude certain groups, according to a report based on the workshop. Some commenters warned that algorithms can deny people opportunities “based on the actions of others.”
In one example, a credit-card company lowered some customers’ credit limits because other people who had shopped at the same stores had a history of late payments.
But when applied differently, other workshops participants noted, big data can be a boon to low-income communities. For example, some companies have compiled and analyzed publicly available data to calculate credit scores for people who previously did not have one.
“Thus, consumers who may not have access to traditional credit, but, for instance, have a professional license, pay rent on time, or own a car, may be given better access to credit than they otherwise would have,” the report says.
There’s no question that algorithms can help humans make difficult decisions more efficiently and accurately. Big data has the power to improve lives, and it often does. But absent a human touch, its single-minded efficiency can further isolate groups that are already at society’s margins.