Future Smartphones Will Tell You What’s Killing Your Plants
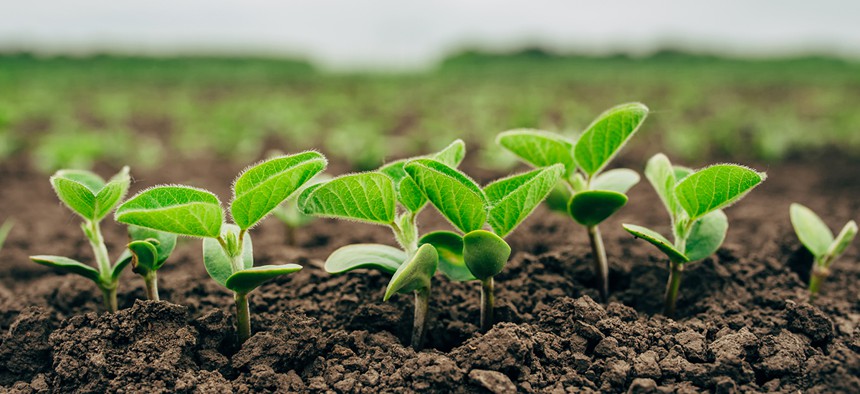
AVANGARD Photography/Shutterstock.com
By amassing a huge library of leaf images, scientist are training computers to diagnose the diseases that threaten our food supply.
A farmer in the Philippines walks through his rice paddies and sees worrying orange smears on his crops. Another in Tanzania detects white blotches on the leaves of her cassava. Worried, they hold up their cellphones, snap pictures and instantly find out whether their plants are diseased, what the infections are and what to do about them.
Here is the future that David Hughes envisions, and it’s slightly closer to reality. Hughes and his colleagues, Sharada Mohanty and Marcel Salathé at the Swiss Federal Institute of Technology in Lausanne, fed a computer with images of 54,000 leaves, and allowed it to learn the features that signify disease. It churned out an algorithm that could diagnose 26 different diseases in the foliage of 14 crops with almost perfect accuracy—99.35 percent.
Admittedly, this was all done using photos taken under clear lighting and standardized backgrounds. Under realistic conditions, the algorithm isn’t anywhere near as good.
But the team thinks that’s just a matter of giving it a broad enough set of images to learn from.
“It’s proof that we can classify very complicated pathology on leaf surfaces automatically,” says Hughes. “There’s no human that can do this. If you’re an expert on tomatoes, you could do tomato diseases, but you couldn’t do all the 26 ones that we used.”
Hughes knows plant diseases. As a kid growing up in Dublin, he could see mounds of barren soil where potatoes had succumbed to blight 150 years earlier, causing famine and social upheaval. As an adult, he has become increasingly worried that plant diseases are neglected and potentially apocalyptic.
Around 90 percent of the world’s calories come from 15 types of crops, which are planted in sprawling, inbred monocultures that are vulnerable to disease. If severe fungal epidemics simultaneously hit just the five most important of these—rice, maize, wheat, potatoes and soybean — 60 percent of the world’s population wouldn’t have enough food.
Compounding the problem, plant pathologists—experts in botanical contagions—are in increasingly short supply. As the old guard retires, so too does the knowledge locked in their heads.
Hughes tried to mitigate those losses by creating PlantVillage—a botanical version of Quora where people around the world can ask each other for agricultural advice. If experts are in decline, perhaps farmers can tap into each other’s expertise.
It’s a nice idea, but while traffic to the site is steadily increasing, most of the replies come from Hughes and his team.
So Hughes has been looking for ways of automating the act of diagnosing plant diseases. First, he needed images, and so he took his team to experimental farms in Ghana where researchers had deliberately inoculated crops with specific pathogens; that way, they could be absolutely sure that they knew the illness represented in whatever photos they took. They captured around 100,000 images, all taken in bright daylight and against a uniform background.
Salathé then fed half the photos into an artificial neural network—a computer system that mimics the interactions between neurons in a brain, and can learn to recognize and classify large sets of images. The network used some of the leaf images to build a program that could recognize any diseases upon them, and then tested that program on the remaining pictures. At its best, it had an accuracy of 99.35 percent.
Other teams have tried similar approaches, but only with one crop or one disease, says Salathé. “Our approach works with 12 crops and 26 diseases. We don’t tell the computer about whether there’s Phytophthora or whether it should check for gray spots. We let these networks figure out, on their own, which features are important for which plants.”
Running the neural network takes a huge amount of computing power, but once the program is built, it can run on a smartphone. And since a growing proportion of the world’s population has mobile broadband access—69 percent at the end of last year, and climbing—the team is hopeful that an app could prove valuable to farmers in the developing world, who need it most.
Other diagnostic apps have been developed, but they either offer an encyclopedias of images, or put you in touch with an expert. None are automatic.
But Janna Beckerman from Purdue University is skeptical about how useful automatic apps can be.
“With plant diseases, I can’t just look at leaves and symptoms,” she says. “I need to go to a microscope. Sometimes, I need to culture things. There are apps, but we always caution people that they need to follow up with a laboratory diagnosis.”
Judy Payne from the U.S. Agency for International Development is more enthusiastic.
“I’m impressed by the potential to help farmers identify hard-to-diagnose plant diseases,” she says. “While this technology is no substitute for the careful eyes of trained plant pathologists, it can go a long way to alleviate the challenges of distance and access faced by many farmers in the developing world.”
Still, she adds, the “technology needs to be well-tested and adapted to rural settings.” Indeed, when the team tested their program on more realistic images, taken from books, scientific papers, and other sources, its accuracy fell to just 31 percent. When they told the program what the crop was, something that real farmers would obviously know, its accuracy rose back to 45 percent.
That doesn’t sound great, but Salathé notes that a random guess would be accurate just 2 percent of the time. And “the best comparison is against a human,” says Hughes. “Could one human get better than 45 percent on classifying 26 diseases on 14 crops? Likely no. A whole department of experts with different capabilities might, but our approach intends to give a grower in Kent, Kentucky, or Kenya that department-level diagnosis.”
The team have made their image library free to access, and they hope that computer scientists will help to devise better algorithms. And they’re working hard to gather more images—of more crops plagued by many strains of various diseases, at different points of the infection cycle, under a range of lighting conditions and backgrounds. That’s the key, Salathé says. “Currently, the algorithm doesn’t have the chance to appreciate the diversity that’s out there. All we need to do now is to add more images, and the accuracy will go up.”
Hughes has teams of photographers snapping shots of rice in the Philippines and cassava in Tanzania.
“I work mainly on cassava, and there alone the techniques could benefit tens of millions of farmers who are badly affected by mosaic and brown streak diseases,” says James Legg from the Consultative Group for International Agricultural Research, and one of Hughes’ collaborators. “They cause annual losses in Africa of more than $1 billion.”
“We are aiming for 3 million images within three years,” says Hughes. “We’ll make them open-access. And if you want to use our images, your code has to be open-access.”
“I see great potential for use of this automatic disease diagnosis by farmers worldwide,” says Saskia Hogenhout from the John Innes Centre. “Moreover, I am sure people will volunteer to contribute images of diseases and pests to the public database making it useful for plant-health agencies to control disease and pest invasions globally.”
NEXT STORY: The money factory needs a CTO