The Promise of Terrapattern: The Visual Search Engine for Satellite Imagery
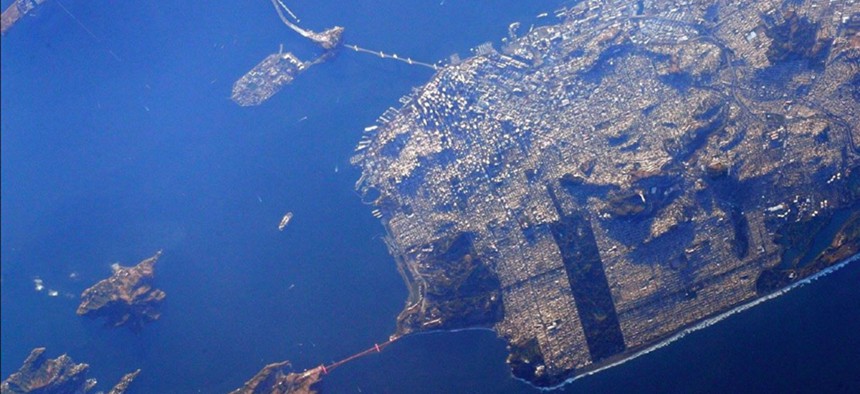
Astronaut Scott Kelly snapped this photo of the San Francisco peninsula from the International Space Station in 2015. NASA
Finding empty pools for skateboarding has never been so easy.
Sometimes, a new tool comes into the world that is so expansively, obviously useful that you can’t do anything but sit back and think: wow.
For me, at least, that’s Terrapattern, a visual search engine for satellite imagery, released this week by a team of artists and geographers at Carnegie Mellon University. It is Google’s “reverse image-search” tool for maps, basically: Click on a spot you find interesting, and Terrapattern will show you other spots on the map like it.
This means you can find all the cul-de-sacs in Pittsburgh:
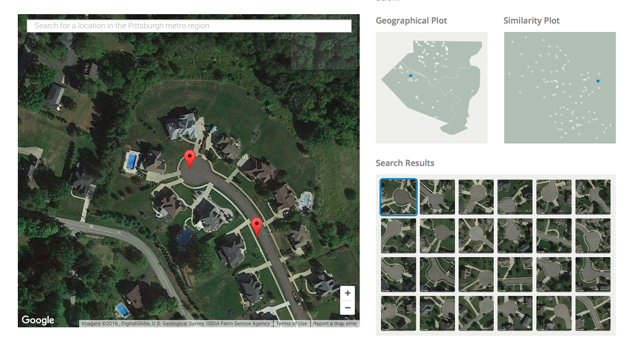
Or all the football fields:
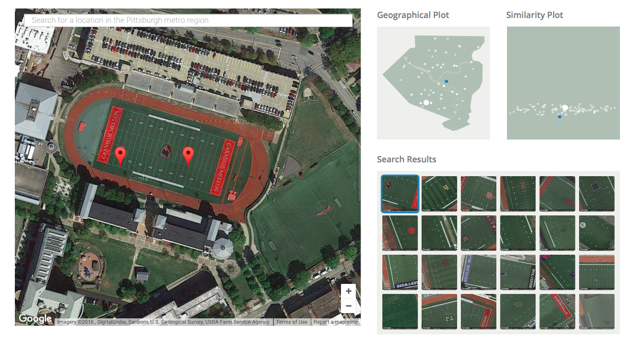
Or all the solar panels in New York City. Or all the bridges in the Bay Area.
“One of our friends is using it to find disused swimming pools for guerilla skateboarding,” writes the Terrapattern team on their site. They built the tool for “discovering ‘patterns of interest’ in unlabeled satellite imagery,” they say, a way to explore “the unmapped and the unmappable.”
Right now, Terrapattern only covers four American cities: Pittsburgh, Detroit, San Francisco and New York City. Terrapattern is so computing-hungry that it is effectively a proof of concept right now, at least for a team of artists working with less than $35,000. Each metro region takes about 10 gigabytes of RAM—not storage, but active memory.
That said, Terrapattern is relatively technically straightforward. It’s constructed from a convolutional neural network and CoverTree, an algorithm that remembers some descriptions and allows the searches to happen quickly.
“It took the neural net about five days to train,” said Golan Levin, an artist and engineer, in an email. Levin led the team that developed Terrapattern. “It was as easy as pointing the neural net at the map tiles,” he said, though the map tiles were associated with place descriptions through OpenStreetMap.
“To be perfectly honest, most of our time was spent moving files around from place to place," he said. "Things get slow when you’re moving hundreds of gigabytes."
Levin and the rest of the Terrapattern team thinks that the tool is especially adept at finding “nonbuilding structures and other forms of otherwise unremarkable soft infrastructure that aren’t usually indicated on maps,” the team writes. Think of those empty swimming pools—or a wind turbine, or an inflated sports dome.
Or for that matter, a bridge damaged by an earthquake. Dale Kunce, who manages international digital mapping at the American Red Cross, told me that Terrapattern likely had applications in humanitarian situations. He imagined a situation where Terrapattern (or software like it) could process a satellite image of a disaster area and produce a “first pass” list of damaged structures. Then, a human editor could come in and cull that list by hand.
“I am not usually impressed by stuff these days, but I was impressed by this,” Kunce said.
He told me it fits into the advance of applying digital maps to disaster relief over the past decade: moving from Google Maps, which included satellite imagery of most places; to OpenStreetMap, which let anyone make and use digital street data for free; to using OpenStreetMap in professional and humanitarian situations.
Now, software like Terrapattern and Facebook’s population-estimating algorithm let volunteers apply their skills of discernment faster and at a greater scale. Estimating storm damage or population centers might be the next step in crowdsourced disaster-relief mapping.
“The most powerful supercomputer in the world is not as good at recognizing things as the human brain," he told me. "No one’s build Watson for satellite imagery."
Levin said it was hard to know when Terrapattern might be ready for humanitarian deployment.
“Currently, our prototype only works in four cities," he said. "San Francisco is not currently suffering from a humanitarian crisis, in any reasonable sense of the word."
But Terrapattern is also not likely the only technology of its kind.
Right now, a number of startups—including Planet Labs and Terra Bella, which is owned by Google—are tossing dozens of small imaging satellites into orbit. They’re doing this because imagery-deciphering technology is expected to mature in the next few years, meaning they could algorithmically read the amount of oil in oil wells for financial firms. Descartes Labs also intends to apply machine learning to imagery in order to estimate agricultural yields.
But there hasn’t been a working product quite like Terrapattern yet—or at least one available to the general public to play around with. It is, as they put it, geospatial-intelligence analysis “for the rest of humanity.”
Terrapattern is an experiment to see if “visual ‘query-by-example’ for satellite imagery might become a part of our everyday future,” they write. “Remember, you saw it here first.”