Government Innovation Award top honors go to NOAA, DOD, Virginia and SAIC
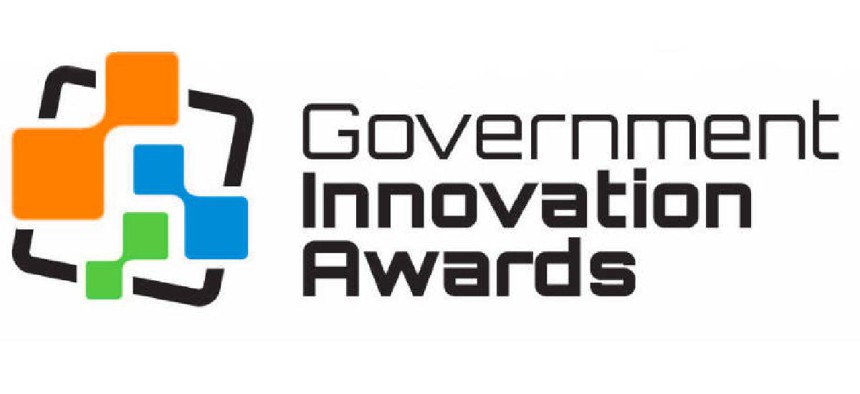
Best in Class recognitions showcase the potential of IoT, advanced analytics and creative public-private partnerships.

The 2019 Government Innovation Awards recognized a broad range of projects, individuals and companies -- 85 winners in all, working at all levels of government. At the Nov. 7 awards dinner, however, three Public Sector Innovations and one Industry Innovator received special Best in Class recognition.
The National Oceanic and Atmospheric Administration’s Big Data Project won the Best in Class award for federal civilian efforts. NOAA's project, which this year completed its experimental phase and is moving into production in 2020, has made massive data sets publicly available at minimal cost to the agency by partnering with multiple cloud service providers.
In the defense sector, top honors went to the Stryker Nuclear Biological Chemical Reconnaissance Vehicle Sensor Suite Upgrade project. The NBCRV SSU team developed a better, smaller and cheaper way to detect chemical, biological, radiological and nuclear threats in the field -- and rolled out a working prototype in just 100 days.
Among state and local efforts, Virginia's Framework for Addiction Analysis and Community Transformation was named Best in Class. A project of the state's Department of Criminal Justice Services, FAACT protects individual privacy while giving state and local officials the ability spot and respond to dangerous trends in opioid abuse in near-real time.
And finally, SAIC won Best in Class among the Industry Innovators for its Battlefield of Internet Things solution. The program incorporates sensors, mobile broadband and networking, cloud computing and other technologies to allow Defense Department organizations to collect and process massive amounts of sensor data, turning it into actionable intelligence.
You can learn more about all this year’s Government Innovation Award winners at GovernmentInnovationAwards.com.
NEXT STORY: Bid Protests Hit 10-Year Low