Statistical Uncertainty Could be Problematic for Evidence-Based Policies, Study Finds
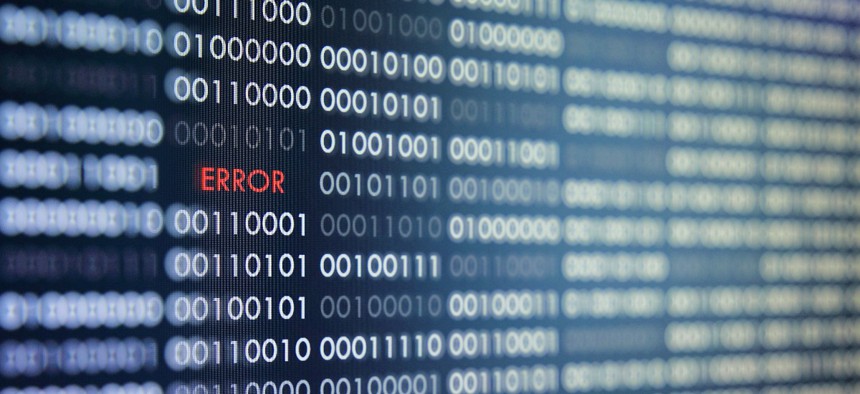
Suebsiri Srithanyarat / EyeEm/Getty Images
Certain privacy measures, as well as general data errors, can skew funding allocations derived from census information.
Statistical uncertainty in data—or random error in a measurement—particularly when it is used to inform funding and policies, can lead to a variety of issues, such as the misallocation of funding.
Academic researchers at Carnegie Mellon University recently conducted a study, called “Policy impacts of statistical uncertainty and privacy,” which examined the implications of statistical uncertainty, data errors and differential privacy on Title I of the Elementary and Secondary Education Act, which provides funding to school districts with high numbers of children from low-income families, finding that the errors led to misallocated funds.
Specifically the study examined the Census Bureau’s adaptation of differential privacy––a tool for preserving privacy within data.
“The idea is that we want what’s called a formal guarantee of privacy,” Ryan Steed, a Ph.D. student at Carnegie Mellon University’s Heinz College, who led the study, told Nextgov. “So, we want to be able to say, in all cases, what’s the probability of some bad thing happening? And generally, what differential privacy does is it says, ‘okay, so if we release some statistics, how much additional information could somebody learn from those statistics about the people who were included in the data used to make those statistics?’ And what we want to sort of promise is that the additional amount of information that you could learn based on whether or not an individual person was included, we want to limit that additional amount of information. So, differential privacy gives us essentially this limit.”
“Differential privacy is one—perhaps currently the most well known and most discussed tools—in an arsenal of tools that statisticians, computer scientists and cryptographers have developed to allow privacy protection, while still making some degree of data analytics possible,” study co-author, Alessandro Acquisti, professor of information technology and public policy at Carnegie Mellon University’s Heinz College, told Nextgov.
They explained that one of the ways to achieve differential privacy or to preserve privacy is to inject noise—adding, for example, a random number to the different statistics—which makes it “hard for someone to infer with any sort of certainty whether or not an individual was included in the database,” Steed said.
An entity may choose to implement differential privacy in its data to help protect the identities of those represented.
The Census Bureau’s 2018 decision to try differential privacy—which was applied to census data for the first time when compiling results from the 2020 count—was viewed as controversial because of the concern of adding noise to census data. States filed a court case against the agency about redistricting and its effects on voting. There were also concerns about how this might affect other programs that rely on census data or impact particular groups.
Steed and Acquisti noted that there is already noise in the census data because of misreporting, such as someone giving the wrong answer or no response, as well as administrative errors.
However, programs such as the Rural Telecommunications Infrastructure Loans and Loan Guarantees, Rural Broadband Access Loans and Loan Guarantees and Telehealth Programs rely on Census Bureau data, for example, for how to distribute funding.
While the focus was on census data, other datasets that the government uses for evidence-based policies with statistical uncertainty could also face similar problems.
However, Steed noted that it is critical to be “thinking about uncertainty when we’re designing policies and thinking about how uncertainty is affecting policy goals.”
Acquisti cautioned against over-generalizing the results of the study, but added, “the results are promising in that they suggest that there are situations where the deploying of differential privacy can be done with some work [for] limited negative downstream effect on policy decisions. And those potential detrimental effects can, in fact, be countered by adopting the policy. So in essence, what we hope is that results such as ours can stimulate more research and more attention by policymakers on research that will attempt to understand context by context, scenario by scenario, the implications of using differential privacy, because on theoretical grounds, differential privacy is a powerful tool.”
One solution is a multi-year average, which provides some stability to the data, according to the study authors.
Steed provided several ideas for the continuation of the study or for further research, particularly looking at the effects of statistical uncertainty on other programs, as well as challenges when multiple datasets must be used.
According to Steed, it would also be informative to look at “the importance in communicating uncertainty to users of census data. Sometimes it’s very difficult, it takes a lot of expertise to figure out how to properly use those margins of error or other uncertainty estimates that are published by the Census Bureau, so ways to better communicate those and make it easier for users of data to appropriately account for them [could be] a good direction for research.”
“If we can figure out ways to address the underlying issue of statistical uncertainty, in general, that much larger issue of data error, then there are positive benefits on the back end. Not only does it make our policy more effective, it also makes it easier for us to have these stronger privacy protections,” he added. “It actually helps out with some of the concerns that those advocates have had back when the Census Bureau first announced their plans and people were really worried about how it might affect all these important use cases.”
Meanwhile, Acquisti discussed future considerations and criticisms, which could potentially help improve this privacy tool.
“Criticisms of differential privacy are fair, we need open debate on these tools,” he said. “That is the only way to a) make them better and b) understand the extent to which they can really be useful. What we hope is that those criticisms do not end up missing the forest for the tree; they may point out a particular problem with differential privacy, but perhaps miss the bigger picture. And we believe that discussing the bigger picture is important in the debate around privacy and data analytics.”
In light of concerns expressed by Denice Ross, chief data scientist at the White House Office of Science and Technology Policy—who urged for the need to better identify disparities in available datasets—both highlight the need for improved data policies and practices, particularly when data is used to inform government programs and policies.