How Algorithms Can Bring Down Minorities' Credit Scores
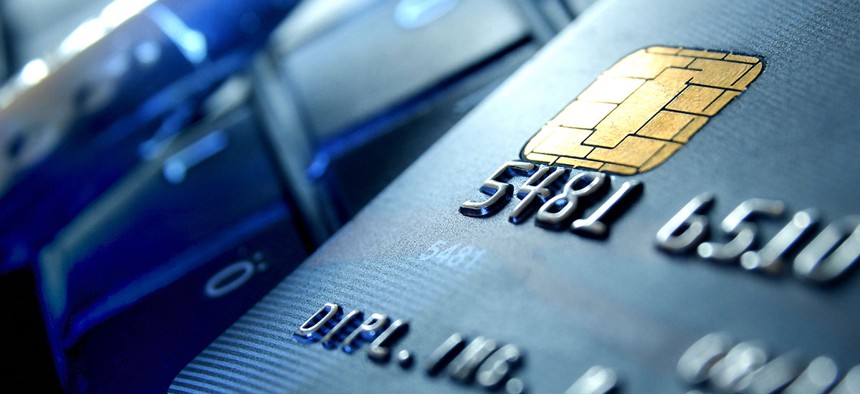
qvist/Shutterstock.com
Analyzing people’s social connections may lead to a new way of discriminating against them.
In one episode of "Black Mirror," the world runs on a rating system. Just like you and your Uber driver rate each other after a ride, every person in the episode’s fictional world scores each other on a five-star scale after every interaction. Each individual’s average score affects their access to basic goods and services, like housing and transportation.
In real life, too, people are followed around by scores and ratings that can grant or deny opportunities—but instead of being determined by other people, they’re assembled by mysterious algorithms. From credit scores to hiring processes, automated systems make decisions based on vast troves of personal information, often without revealing the kinds of information included in the calculus. Some even take into account people’s friends, families and acquaintances to make assumptions about their character traits—which some privacy experts worry could lead to discrimination.
A German company called Kreditech, for instance, asks loan applicants to share information from their social-media networks, which they can comb for details about their friends. Being connected to someone who’s already paid back a loan with the company is “usually a good indicator,” the company’s chief financial officer told Financial Times.
In India and Russia, FICO—the company behind the popular FICO credit scores—is partnering with startups like Lenddo to capture information about users from their cellphones. Lenddo uses locations reported by applicants’ phones to figure out whether they really live and work where they say they do, and then analyzes an applicant’s network to figure out “if they are in touch with other good borrowers—or with people with long histories of fooling lenders,” Bloomberg reports.
The push to consider more types of information can help people who lack credit scores, or who might not have the usual indicators of creditworthiness, access loans and bank accounts that might otherwise be closed off to them.
But the more complex and opaque these powerful algorithms get, the more ways there are for people to be disqualified from job searches and loan applications—and the harder it is to know why.
What’s more, systems that take into account the actions of people’s family and friends risk assigning guilt by association, denying opportunities to someone because of who they’re connected to. They can decrease a person’s chance for upward mobility, based solely on the social group they find themselves in.
Someone living in a low-income community, for example, is likely to have friends and family with similar income levels. It’s more likely someone in their extended network would have a poor repayment history than someone in the network of an upper-middle class white-collar worker—if a scoring algorithm took that fact into account, it might lock out the low-income person just based on his or her social environment.
The Equal Credit Opportunity Act doesn’t allow creditors in the United States to discriminate based on race, color, religion, national origin, sex, marital status, age—but taking into account a person’s network could allow creditors to end-run those requirements.
A 2007 report from the Federal Reserve found blacks and Hispanics had lower credit scores than whites and Asians, and “residing in low-income or predominantly minority census tracts” is a predictor of low credit scores. Because people are likely to have friends and family who live nearby and are the same race, using social networks to rate their creditworthiness could reintroduce factors creditors aren’t allowed to consider.
In an essay published in 2014 by New America’s Open Technology Institute, three privacy researchers—danah boyd, Karen Levy and Alice Marwick—wrote about the potential for discrimination when algorithms examine people’s social connections:
The notion of a protected class remains a fundamental legal concept, but as individuals increasingly face technologically mediated discrimination based on their positions within networks, it may be incomplete. In the most visible examples of networked discrimination, it is easy to see inequities along the lines of race and class because these are often proxies for networked position. As a result, we see outcomes that disproportionately affect already marginalized people.
Preventing algorithmic discrimination is a challenge. It’s not easy to hold companies to the laws that would protect consumers from unfair credit practices, says Danielle Citron, a law professor at the University of Maryland.
“We don’t have hard and fast rules," she said. "It’s the Wild West in some respects.”
The agencies in charge of enforcing the relevant laws—the civil rights division of the Justice Department, the Consumer Financial Protection Bureau and the Federal Trade Commission—have a mixed record of going after companies that violate them, Citron says. And once their reins are handed to President-elect Donald Trump, they may be even less interested in pursuing violators, preferring instead to “let the free market deal with it,” she anticipates.
A look abroad shows how far companies—and even governments—are willing to take scoring. Far beyond the network-based scoring systems in Russia and India, China is now testing a system that would assign Chinese citizens a score that goes far beyond the credit scores we’re used to.
According to The Wall Street Journal, the system takes into account the usual financial factors, but mixes in “social inputs” like adherence to laws (including traffic violations and paying for public transportation), academic honesty and volunteer activity. The system will likely also use online indicators like shopping patterns and interactions with others.
The score could end up determining whether people can access internet services, jobs, education, transportation and various other goods and services—not unlike the universal ratings in "Black Mirror."
U.S. laws would bar such a sweeping government-run system from being put in place here. But private companies, which are subject to different regulations than the federal government, could push closer to such a reality—indeed, credit scores in their current form already affect people’s lives in crucial ways.
“We’re not as far off as we think,” Citron says.