Oak Ridge-Made Software Platform Digitally Twins All U.S. Buildings
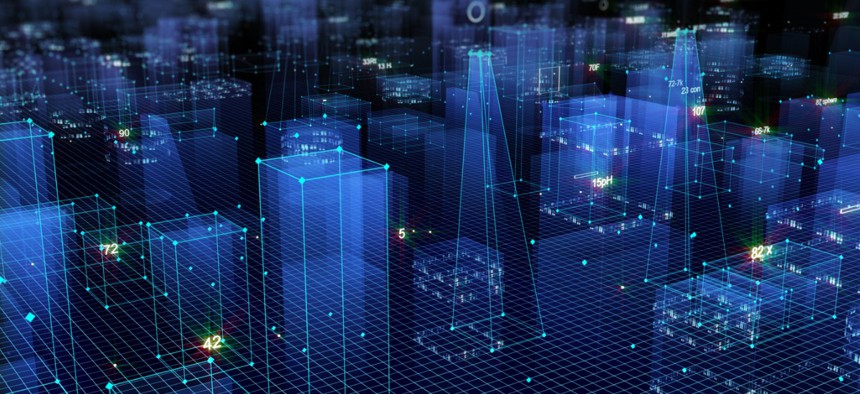
vitacopS/iStock.com
Those involved aim to help improve nationwide decision-making about energy efficiency.
Researchers within the Energy Department’s Oak Ridge National Laboratory built a modeling software suite that uses publicly available data to provide specifics and enable people to fully visualize energy details about every one of America’s 129 million buildings.
That simulation tool—dubbed the Automatic Building Energy Modeling, or AutoBEM—was years in the making. Its creation stemmed in part from the Tennesee-based lab’s longstanding goal to generate digital twins for all of the nation’s structures. Those behind it aim to help home and business owners access thorough information needed to make more actionable decisions around boosting energy efficiency.
“A digital twin is a virtual model of a physical object or system,” Joshua New, a computer scientist and senior research and development staff member at ORNL told Nextgov on Thursday. “This is useful because it allows us to find ways to improve performance more quickly without the risk or expense of conducting experiments in the real world.”
New led the lab’s research team that created AutoBEM and made those models publicly available earlier this year. He shed light on the platform’s production, potential use cases and what’s next for this work.
While technology was there to make it possible, a single resource to quantify the energy makeup of all U.S. buildings didn’t exist before this work kicked off in 2015. But at that time, researchers recognized that an asset like that could help as America works to reach net-zero carbon emissions by 2050. Buildings, according to stats mentioned by ORNL, account for 40% of the United States’ energy consumption and 75% of its electricity.
To make AutoBEM, New and the team explored heaps of available data sources and collaborated with officials who work in the realm of satellite, aerial, street view and LiDAR data. As resources were identified, they had to use or extend algorithms to turn all that data into structure descriptions, and digital footprints of buildings. Essentially, the platform transforms that imagery from satellites, streets and elsewhere into usable insights about the buildings, such as their numbers of floors and windows; the various heating, cooling and ventilation systems they house; and their roof types, among other elements.
With that information, the program also uses high-performance computing to produce building models that can be used to predict technologies that could be implemented to help save energy.
“The easiest way to explain it is—think of a basic mapping service, like Google Maps, then overlay a 3D representation of every building,” New explained. “Any building on the map can be clicked on to bring up an extensive data set about that building’s energy characteristics.”
He and the team turned to supercomputing assets from Oak Ridge and Argonne National Lab’s Leadership Computing Facility for this work, which was funded by the Energy Department’s Office of Electricity. Details about buildings’ makeup and overall energy performance are made accessible, but the tool doesn’t share details about what’s inside of them.
AutoBEM’s software was initially designed in partnership with the Electric Power Board of Chattanooga, New confirmed, with comparison to 15-minute electricity use from each building. The intent was to essentially use these predictions of energy, demand, emissions and cost reductions to inform the design of energy efficiency and demand response programs at U.S. utilities, he noted.
People have been assessing how to best put the platform to use since it was publicly released. Urban planners and energy justice advocates could use AutoBEM to assess entire blocks and neighborhoods, and pinpoint areas that have been historically overlooked in building improvement efforts. New said conversations have also occurred around use cases that involve city-scale energy impact projects, simulation-informed analysis to inform business design, sales and marketing leads—and more. Further, many businesses don’t have abilities to access all the data they could to inform energy efficiency-centered choices.
“What I’m most excited about is the unanticipated use cases and business models this data might unlock—especially for energy and climate change thought leaders,” New added.
The Oak Ridge team can also automatically create a “dynamic archetype,” he noted. That component can select the best building models and calculate floor space multipliers that can represent any geographical region of interest, without the need to simulate every building for a particular area. “This allows one to perform building analysis relevant to their interests without needing a supercomputer,” New said.
Efforts like this one fall into the sphere of urban-scale energy modeling, which the computer scientist added “has become a hot topic” over the last decade. To date, nearly all efforts have involved either local data or sources that are not scalable to the entire country for building-specific models, or representative models that represent groups of buildings, rather than building-specific details.
“What makes this novel approach work is leveraging non-traditional data sources, world-class high-performance computing, and scaling to generate and simulate millions of buildings,” New explained.
Looking ahead, he and his group plan to work with the private sector to make these models, building data, and analysis more useful and accessible.
“ORNL’s team has proposed providing annual improvements to these models with the aim of moving toward ‘investment-grade models,’” New said. “Achieving that would allow the models’ predicted savings to be taken to a bank to secure third party financing for implementing energy-efficient technologies in buildings. Market penetration at speed and scale.”