Google’s Powerful AI Spotlights a Human Cognitive Glitch: Mistaking Fluent Speech for Fluent Thought
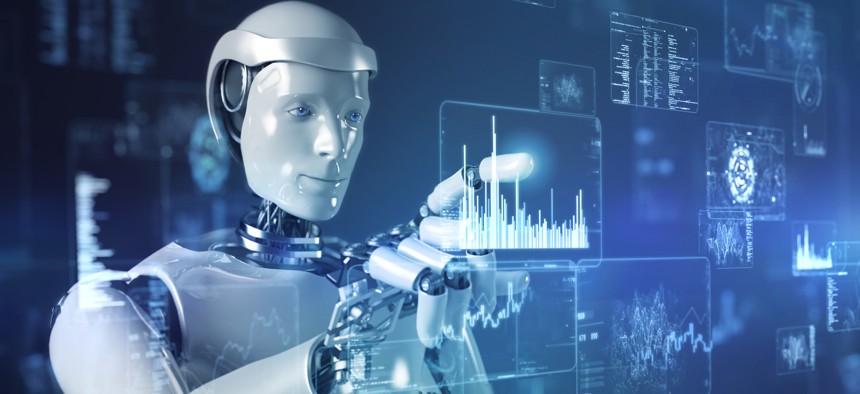
Yuichiro Chino/Getty
Learning language does not mean artificial intelligence is conscious, according to researchers.
When you read a sentence like this one, your past experience tells you that it’s written by a thinking, feeling human. And, in this case, there is indeed a human typing these words: [Hi, there!] But these days, some sentences that appear remarkably humanlike are actually generated by artificial intelligence systems trained on massive amounts of human text.
People are so accustomed to assuming that fluent language comes from a thinking, feeling human that evidence to the contrary can be difficult to wrap your head around. How are people likely to navigate this relatively uncharted territory? Because of a persistent tendency to associate fluent expression with fluent thought, it is natural – but potentially misleading – to think that if an AI model can express itself fluently, that means it thinks and feels just like humans do.
Thus, it is perhaps unsurprising that a former Google engineer recently claimed that Google’s AI system LaMDA has a sense of self because it can eloquently generate text about its purported feelings. This event and the subsequent media coverage led to a number of rightly skeptical articles and posts about the claim that computational models of human language are sentient, meaning capable of thinking and feeling and experiencing.
The question of what it would mean for an AI model to be sentient is complicated (see, for instance, our colleague’s take), and our goal here is not to settle it. But as language researchers, we can use our work in cognitive science and linguistics to explain why it is all too easy for humans to fall into the cognitive trap of thinking that an entity that can use language fluently is sentient, conscious or intelligent.
Using AI to generate humanlike language
Text generated by models like Google’s LaMDA can be hard to distinguish from text written by humans. This impressive achievement is a result of a decadeslong program to build models that generate grammatical, meaningful language.
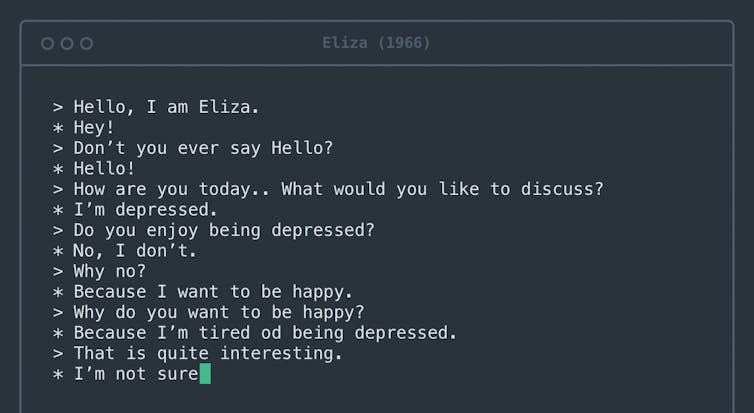
Early versions dating back to at least the 1950s, known as n-gram models, simply counted up occurrences of specific phrases and used them to guess what words were likely to occur in particular contexts. For instance, it’s easy to know that “peanut butter and jelly” is a more likely phrase than “peanut butter and pineapples.” If you have enough English text, you will see the phrase “peanut butter and jelly” again and again but might never see the phrase “peanut butter and pineapples.”
Today’s models, sets of data and rules that approximate human language, differ from these early attempts in several important ways. First, they are trained on essentially the entire internet. Second, they can learn relationships between words that are far apart, not just words that are neighbors. Third, they are tuned by a huge number of internal “knobs” – so many that it is hard for even the engineers who design them to understand why they generate one sequence of words rather than another.
The models’ task, however, remains the same as in the 1950s: determine which word is likely to come next. Today, they are so good at this task that almost all sentences they generate seem fluid and grammatical.
Peanut butter and pineapples?
We asked a large language model, GPT-3, to complete the sentence “Peanut butter and pineapples___”. It said: “Peanut butter and pineapples are a great combination. The sweet and savory flavors of peanut butter and pineapple complement each other perfectly.” If a person said this, one might infer that they had tried peanut butter and pineapple together, formed an opinion and shared it with the reader.
But how did GPT-3 come up with this paragraph? By generating a word that fit the context we provided. And then another one. And then another one. The model never saw, touched or tasted pineapples – it just processed all the texts on the internet that mention them. And yet reading this paragraph can lead the human mind – even that of a Google engineer – to imagine GPT-3 as an intelligent being that can reason about peanut butter and pineapple dishes.
The human brain is hardwired to infer intentions behind words. Every time you engage in conversation, your mind automatically constructs a mental model of your conversation partner. You then use the words they say to fill in the model with that person’s goals, feelings and beliefs.
The process of jumping from words to the mental model is seamless, getting triggered every time you receive a fully fledged sentence. This cognitive process saves you a lot of time and effort in everyday life, greatly facilitating your social interactions.
However, in the case of AI systems, it misfires – building a mental model out of thin air.
A little more probing can reveal the severity of this misfire. Consider the following prompt: “Peanut butter and feathers taste great together because___”. GPT-3 continued: “Peanut butter and feathers taste great together because they both have a nutty flavor. Peanut butter is also smooth and creamy, which helps to offset the feather’s texture.”
The text in this case is as fluent as our example with pineapples, but this time the model is saying something decidedly less sensible. One begins to suspect that GPT-3 has never actually tried peanut butter and feathers.
Ascribing intelligence to machines, denying it to humans
A sad irony is that the same cognitive bias that makes people ascribe humanity to GPT-3 can cause them to treat actual humans in inhumane ways. Sociocultural linguistics – the study of language in its social and cultural context – shows that assuming an overly tight link between fluent expression and fluent thinking can lead to bias against people who speak differently.
For instance, people with a foreign accent are often perceived as less intelligent and are less likely to get the jobs they are qualified for. Similar biases exist against speakers of dialects that are not considered prestigious, such as Southern English in the U.S., against deaf people using sign languages and against people with speech impediments such as stuttering.
These biases are deeply harmful, often lead to racist and sexist assumptions, and have been shown again and again to be unfounded.
Fluent language alone does not imply humanity
Will AI ever become sentient? This question requires deep consideration, and indeed philosophers have pondered it for decades. What researchers have determined, however, is that you cannot simply trust a language model when it tells you how it feels. Words can be misleading, and it is all too easy to mistake fluent speech for fluent thought.
Kyle Mahowald, Assistant Professor of Linguistics, The University of Texas at Austin College of Liberal Arts and Anna A. Ivanova, PhD Candidate in Brain and Cognitive Sciences, Massachusetts Institute of Technology (MIT)
This article is republished from The Conversation under a Creative Commons license. Read the original article.