Federal Networks Under Attack: How Artificial Intelligence Will Save the Day
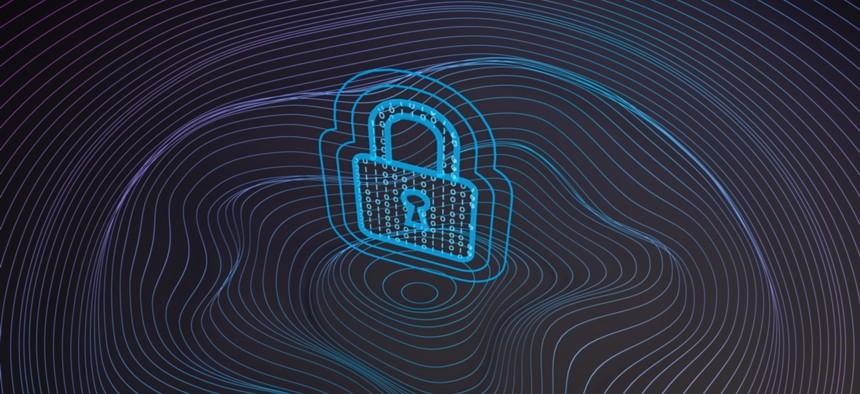
SCH KK/Shutterstock.com
It's time to stop being a step behind hackers.
There aren’t enough hours in the day for the average cybersecurity analyst to deal with every threat. Analysts face about 300 security alerts per day, often as many as 400 to 500. It takes about five minutes to analyze each one, which adds up to 25 hours in a day. Our frontline defenses are outgunned from the start.
How can federal and state IT teams protect sensitive government data without hiring tens of thousands of people? One possible solution is artificial intelligence. AI can be used to quickly process and analyze information, giving analysts better insight into which alerts are actionable and which can be ignored. That kind of information can drastically cut down the triage process, giving analysts more time to focus on the most critical threats.
To fend off these attacks, two major problems need to be addressed: massive amounts of data, and static threat detection.
Let’s take data first: A large organization like a government agency may have upwards of one to two petabytes of traffic on its systems every day. This amount of information takes days for a traditional CPU to process but can be done in minutes using GPUs, which use massive parallelization to process data and run analytics.
Beyond that, the architecture that flags these alerts is often running on pre-built and quickly outdated signatures used to analyze and verify traffic coming in and out of the network. Unique signatures are assigned to known threats so that if they enter the network, they’re easily identified and rooted out. This method works well when you know what you’re looking for, but unknown threats are often the biggest problems. Pre-built signatures can’t detect increasingly sophisticated malware being authored by an increasing number of threats globally.
AI can play a role in solving this problem as well. By utilizing deep learning and machine learning, threat detection can evolve, and processes can be tailored around a specific network through behavioral analysis. This is done by deploying AI processes to learn how users and machines on the network typically act with other network assets. The GPU-powered models can, in near real-time, detect distributional traffic shifts, anomalous patterns of network behavior and creative attacks as they originate.
Companies like Booz Allen Hamilton are already working with federal agencies to take on the monumental task of making government networks more secure through the use of AI. They’ve created a detection model deployed on GPU architecture that uses neural networks and Bayesian statistical models—which determine the probability of an event based on prior knowledge—to construct comprehensive cyber-alerting solutions that are specifically tuned for each observed network. That means the system grows stronger the more it’s used because it’s learning from each interaction.
Without AI augmentation to assist human analysts, agencies will be a step behind hackers, constantly trying to update their security to face the next big threat. But by utilizing GPU-powered technology, they’ll be ready for whatever comes, building a more resilient shield along the way.
Bartley Richardson is a senior data scientist for AI infrastructure at NVIDIA.
NEXT STORY: 3 Steps to a More Secure Federal Supply Chain