Why Government Agencies Need to Incorporate Explainable AI in 2021
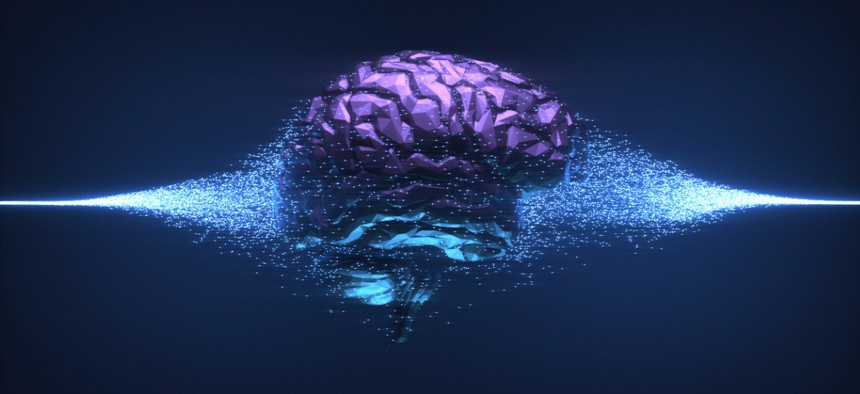
Just_Super/iStock.com
Consumers want reassurance about ethical use and fairness related to AI.
In a world fueled by digital data, the use of artificial intelligence is prolific—from the automation of human processes to discovering hidden insights at scale and speed. Machines can do many tasks far more efficiently and reliably than humans, resulting in everyday life that increasingly resembles science fiction. This inevitably sparks concern about controls—or lack thereof—to inspect and ensure these advanced technologies are used responsibly.
Consumers want reassurance about ethical use and fairness related to AI. Businesses need to mitigate the risk of unintended consequences when employing these advanced, complex solutions. Enter: Explainable AI, or XAI, an attempt to create transparency in the “black box” of artificial intelligence.
Can you confidently answer the simple questions below about your current AI solutions?
- Why did the AI model make a specific decision or prediction?
- When the result is unexpected, why did the model pick an alternate choice?
- How much confidence can be placed in the AI model results?
What is Explainable AI?
Think of it as a two-step process: first, interpretability, the ability to interpret an AI model, and second, explainability, to be able to explain it in a way humans can comprehend. Interpretability is the extent to which an AI model’s decisions can be comprehended (understanding of the raw mechanics of the model) and the ability to anticipate model results.
Explainability takes this further and where a model’s decision or prediction can be made transparent and communicated to humans. In other words, a human would be able to understand why a decision was made without being an expert in advanced math.
The Expanding Need for XAI Within Government
The increase in the use of AI in the last decade has U.S. agencies monitoring the debate about AI ethics and seeking signs of potential regulation. There is considerable public debate on the ethical use of AI and federal departments such as the Health and Human Services and Defense departments are solidifying a commitment to “Ethical, Trustworthy AI Use and Development” and “AI Ethical Principles.”
Transparency in how AI models work their explainability is a key part of monitoring and ensuring compliance with these ethical principles. The link between AI model explainability and AI ethics was also reinforced recently when the National Institute of Standards and Technology proposed four principles for explainable artificial intelligence: explanation, meaningful, explanation accuracy and knowledge limits. NIST recognizes the “challenges of designing explainable AI systems” and indicates these four principles are “heavily influenced by considering the AI system’s interaction with the human recipient of the information.” However, NIST believes XAI “contributes to the safe operation and trust of multiple facets of complex AI systems.”
But barriers to adopting XAI remain, including:
- Increased Initial Investment: Using an XAI approach to AI model development may increase the amount of initial investment to support model transparency requirements. It could also deter the selection of an advanced technique that provides superior results but cannot be easily explained. Alternatively, the potential risk of unintended, negative outcomes using a ‘black box’ AI approach could result in much higher costs in the long term to remediate.
- Intellectual Property: Some of the latest research has shown that as the explainability of a machine learning model increases, the security of that model can decrease. Given full explainability, a model can be reverse-engineered or recreated but can pose a threat to the intellectual property of the technologies involved in its original creation. Additionally, such information can expose the model to hacking or hijacking. Mitigating this risk through the ability to explain the model and transparency is a possibility–however, this level of information is only shared with a limited audience.
While there are many benefits to consumers and businesses alike when leveraging AI, the complexity of AI models can make it difficult to answer questions about how a decision was made or an action taken. To regulate AI both informally or formally, we must be able to understand and explain it. To mitigate risk of legal and moral issues when employing AI solutions, government leaders must consider XAI as part of any plan for AI ethics.
Claire Walsh is the vice president of engineering at Excella Inc.