Artificial Intelligence is Good at Less Exciting Military Roles Too
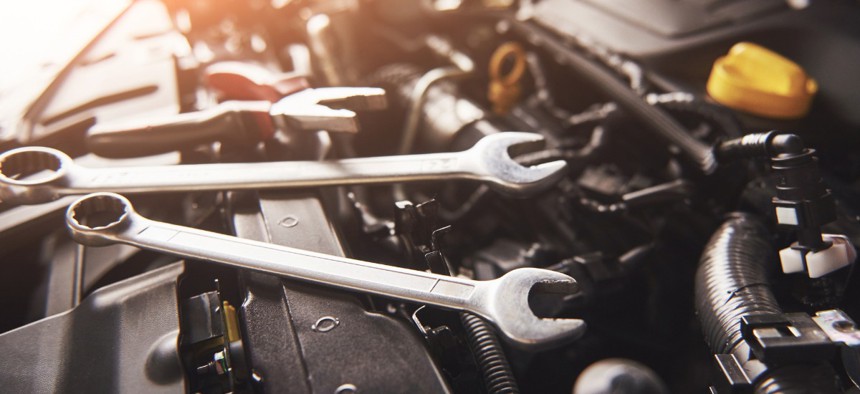
standret/istockphoto.com
Maintenance is critical in the military, and this is a good example of how AI can be used right now to help improve operations.
I’ve received a lot of feedback regarding my last few columns about drones, simulations and new uses for artificial intelligence. My most recent column about creating an AI that could task the kind of swarm intelligence found in bee colonies to do some amazing things, like controlling weather satellites, in particular generated a lot of buzz.
Much of the feedback I received was from federal agencies and IT companies working on futuristic AI programs. I’ll probably be highlighting some of them in the near future. But there was also a note from a company called Hypergiant about how they were working with the Army’s Robotic Combat Vehicle program not for some advanced combat project, but simply using AI to help with predictive maintenance tasks.
Founded in 2018, Hypergiant is an enterprise AI company focused on developing world-changing technology to solve the world's biggest problems in the areas of space, defense and critical infrastructure.
Maintenance is critical in the military, and this is a good example of how AI can be used right now to help improve operations. It may seem a bit mundane, but employing AI for routine tasks could have a big impact in the long run. I talked with General Manager of Space and Defense for Hypergiant Industries Quentin Donnellan about how AI and other similar technologies like machine learning can be employed to increase efficiency and improve operations in military settings.
Nextgov: How can AI be used for mundane tasks like setting up vehicle maintenance schedules that are normally performed by humans?
Donnellan: Our goal is to make operations for vehicle sustainment simply much more efficient. There is so much time wasted by our military men and women on very tedious, very manual and candidly very inefficient maintenance routines. Success for us means that military vehicle fleets are more effectively utilized, and our men and women in uniform are engaged in much higher cognitive tasks, which will lead to a more capable and cost-effective defense force.
Nextgov: Even though it’s not as headline grabbing as the AI inside an autonomous military vehicle, could having an AI controlling the maintenance of those vehicle fleets go a long way toward ensuring that they have a long and useful lifespan?
Donnellan: That’s right. Autonomous vehicles have an immediate need to leverage AI to respond to environmental stimuli and perform real-time actions, like avoiding an obstacle or navigating to an item of interest. But over time, we can also use AI and machine learning to discover patterns in the data. Those patterns may be related to the performance or material condition of the vehicle, and if so, we can use them to inform maintenance schedules.
Nextgov: And why specifically is predictive maintenance so important when it comes to the safety of autonomous vehicles?
Donnellan: Well, the obvious answer is that by more accurately predicting when a critical component will fail, you can directly reduce the number of vehicle failures. If we’re talking about a drone, that should mean fewer crashes, which may reduce injuries. If we’re talking about passenger vehicles, predictive systems could potentially recognize a tire failure and reduce the chance of a serious accident.
There’s value here that’s not specific to autonomous vehicles, but there is also a financial aspect to it that I think hits autonomous vehicles especially hard. These vehicles may operate in hard-to-reach or sparsely populated locations. Equipment failure may require an expensive human intervention that may not be addressed for a long time, so predictive maintenance can really help reduce the number of times that a human repair crew has to go on-site to fix a broken robot.
Nextgov: So how does your AI handle predictive maintenance? How does it work and what factors or databases of information does it consider?
Donnellan: It really varies on a case-by-case basis. In general though, there are a couple of layers to a maintenance system like sensors on the vehicle collecting data about the vehicle like pressure, temperature and voltage. That data might be processed by ML algorithms running on the vehicle itself which also sends alerts to a local operator for immediate response if needed. That data is then also sent to a hub where different ML algorithms are watching for long-running trends.
At the very highest level, data from all vehicles in a fleet are collected and aggregated in cloud-based data stores and you may have operators marshalling sustainment activities based on a holistic view of the fleet’s status. This may include proactively ordering new parts or consumables in response to insights from the system.
Nextgov: In terms of vehicle parts, can the maintenance AI also have an impact on the military’s supply chain?
Donnellan: Yes, and candidly, this is where predictive maintenance has the largest business impact. Also, if you’ve established a model for predicting supply chain needs based on vehicle conditions, we can then simulate a range of scenarios for supply chain demand based on hypothetical fleet conditions. For example, we may want to simulate an event where vehicles experience an exceptionally hot environment for an extended period. Will that affect my predictive models, and if so, what does that mean from a supply chain perspective? Those sort of insights are incredibly powerful.
Nextgov: Most AI needs to be trained, and this is especially true of machine learning. What is the process like for getting your AI up to speed and skilled in predictive maintenance?
Donnellan: This is perhaps one of the most challenging, if not the most challenging, aspect of machine learning, because the models that generate predictive insights are only as good as the data they were trained on. It’s a constant fight to equip models with accurately labeled data and replacing old models with newly trained ones as often as necessary.
Updating ML algorithms that are running on the vehicle itself can be tricky because those vehicles may not always be connected to the internet or a network where software developers send out updates. Much of the work we do centers around building a digital infrastructure that enables ML engineers to re-train and re-publish models even out to edge deployed computers, like those running on autonomous vehicles.
Another key element in all of this is monitoring the data collected by the vehicle sensors and verifying that it matches the data the ML models were trained on. For the military, you also must understand that any software updates that get deployed to secure systems must go through an auditing process. Finding a way to rapidly deploy retrained ML models that doesn’t violate security workflows can be a real challenge.
Nextgov: That all sounds great, but just to be clear, ultimately the AI is not replacing human technicians, correct? It’s doing a lot of the backend work, much of the tedious stuff, but ultimately handing over repair responsibility to a human?
Donnellan: Yes, at the end of the day, the maintenance performed on the vehicle stays mostly the same as it always has, it’s just better informed. AI might be able to tell you when you need to change the oil in your car, but it can’t change the oil for you—or at least, that’s a different system altogether!
Nextgov: If you are able to prove the value of AI predictive maintenance for autonomous vehicles in the military, what are the next steps? Is there value beyond just a vehicle maintenance program?
Donnellan: Looking to the future, once we’ve proven the value for integrating AI and ML into vehicle maintenance, we’ll want to implement predictive techniques into any piece of equipment that degrades over time.
John Breeden II is an award-winning journalist and reviewer with over 20 years of experience covering technology. He is the CEO of the Tech Writers Bureau, a group that creates technological thought leadership content for organizations of all sizes. Twitter: @LabGuys