How Does ChatGPT Differ From Human Intelligence?
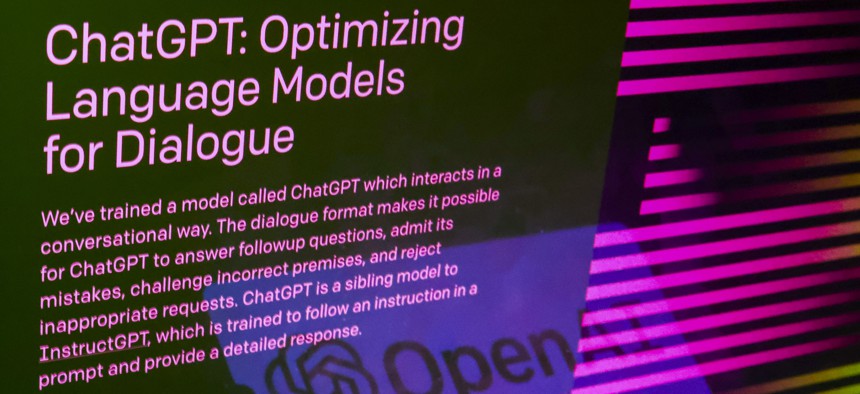
NurPhoto /Getty
The new software learns differently than people.
If ChatGPT sounds like a human, does that mean it learns like one, too? And just how similar is the computer brain to a human brain?
ChatGPT, a new technology developed by OpenAI, is so uncannily adept at mimicking human communication that it will soon take over the world—and all the jobs in it. Or at least that’s what the headlines would lead the world to believe.
In a February 8 conversation organized by Brown University’s Carney Institute for Brain Science, two Brown scholars from different fields of study discussed the parallels between artificial intelligence and human intelligence. The discussion on the neuroscience of ChatGPT offered attendees a peek under the hood of the machine learning model-of-the-moment.
Ellie Pavlick is an assistant professor of computer science and a research scientist at Google AI who studies how language works and how to get computers to understand language the way that humans do.
Thomas Serre is a professor of cognitive, linguistic, and psychological sciences and of computer science who studies the neural computations supporting visual perception, focusing on the intersection of biological and artificial vision. Joining them as moderators were Carney Institute director and associate director Diane Lipscombe and Christopher Moore, respectively.
Pavlick and Serre offered complementary explanations of how ChatGPT functions relative to human brains, and what that reveals about what the technology can and can’t do. For all the chatter around the new technology, the model isn’t that complicated and it isn’t even new, Pavlick said. At its most basic level, she explained, ChatGPT is a machine learning model designed to predict the next word in a sentence, and the next word, and so on.
This type of predictive-learning model has been around for decades, said Pavlick, who specializes in natural language processing. Computer scientists have long tried to build models that exhibit this behavior and can talk with humans in natural language. To do so, a model needs access to a database of traditional computing components that allow it to “reason” overly complex ideas.
What is new is the way ChatGPT is trained, or developed. It has access to unfathomably large amounts of data—as Pavlick said, “all the sentences on the internet.”
“ChatGPT, itself, is not the inflection point,” Pavlick said. “The inflection point has been that sometime over the past five years, there’s been this increase in building models that are fundamentally the same, but they’ve been getting bigger. And what’s happening is that as they get bigger and bigger, they perform better.”
What’s also new is the way that the ChatGPT and its competitors are available for free public use. To interact with a system like ChatGPT even a year ago, Pavlick said, a person would need access to a system like Brown’s Compute Grid, a specialized tool available to students, faculty, and staff only with certain permissions, and would also require a fair amount of technological savvy. But now anyone, of any technological ability, can play around with the sleek, streamlined interface of ChatGPT.
DOES CHATGPT REALLY THINK LIKE A HUMAN?
Pavlick said that the result of training a computer system with such a massive data set is that it seems to pick up general patterns and gives the appearance of being able to generate very realistic-sounding articles, stories, poems, dialogues, plays, and more. It can generate fake news reports, fake scientific findings, and produce all sorts of surprisingly effective results—or “outputs.”
The effectiveness of their results have prompted many people to believe that machine learning models have the ability to think like humans. But do they?
ChatGPT is a type of artificial neural network, explained Serre, whose background is in neuroscience, computer science, and engineering. That means that the hardware and the programming are based on an interconnected group of nodes inspired by a simplification of neurons in a brain.
Serre said that there are indeed a number of fascinating similarities in the way that the computer brain and the human brain learn new information and use it to perform tasks.
“There is work starting to suggest that at least superficially, there might be some connections between the kinds of word and sentence representations that algorithms like ChatGPT use and leverage to process language information, vs. what the brain seems to be doing,” Serre said.
For example, he said, the backbone of ChatGPT is a state-of-the-art kind of artificial neural network called a transformer network. These networks, which came out of the study of natural language processing, have recently come to dominate the entire field of artificial intelligence. Transformer networks have a particular mechanism that computer scientists call “self-attention,” which is related to the attentional mechanisms that are known to take place in the human brain.
Another similarity to the human brain is a key aspect of what has enabled the technology to become so advanced, Serre said. In the past, he explained, training a computer’s artificial neural networks to learn and use language or perform image recognition would require scientists to perform tedious, time-consuming manual tasks like building databases and labeling categories of objects.
Modern large language models, such as the ones used in ChatGPT, are trained without the need for this explicit human supervision. And that seems to be related to what Serre referred to as an influential brain theory known as the predictive coding theory. This is the assumption that when a human hears someone speak, the brain is constantly making predictions and developing expectations about what will be said next.
While the theory was postulated decades ago, Serre said that it has not been fully tested in neuroscience. However, it is driving a lot of experimental work at the moment.
“I would say, at least at those two levels, the level of attention mechanisms at the core engine of this networks that are consistently making predictions about what is going to be said, that seems to be, at a very coarse level, consistent with ideas related to neuroscience,” Serre said during the event.
There has been recent research that relates the strategies used by large language models to actual brain processes, he noted: “There is still a lot that we need to understand, but there is a growing body of research in neuroscience suggesting that what these large language models and vision models do [in computers] is not entirely disconnected with the kinds of things that our brains do when we process natural language.”
On a darker note, in the same way that the human learning process is susceptible to bias or corruption, so are artificial intelligence models. These systems learn by statistical association, Serre said. Whatever is dominant in the data set will take over and push out other information.
“This is an area of great concern for AI, and it’s not specific to languages,” Serre said. He cited how the overrepresentation of Caucasian men on the internet has biased some facial recognition systems to the point where they have failed to recognize faces that don’t appear to be white or male.
“The systems are only as good as the training data we feed them with, and we know that the training data isn’t that great in the first place,” Serre said. The data also isn’t limitless, he added, especially considering the size of these systems and the voraciousness of their appetite.
The latest iteration of ChatCPT, Pavlick said, includes reinforcement learning layers that function as guardrails and help prevent the production of harmful or hateful content. But these are still a work in progress.
“Part of the challenge is that… you can’t, give the model a rule—you can’t just say, ‘never generate such-and-such,'” Pavlick said. “It learns by example, so you give it lots of examples of things and say, ‘Don’t do stuff like this. Do do things like this.’ And so it’s always going to be possible to find some little trick to get it to do the bad thing.”
NOPE, CHATGPT DOESN’T DREAM
One area in which human brains and neural networks diverge is in sleep—specifically, while dreaming. Despite AI-generated text or images that seem surreal, abstract, or nonsensical, Pavlick said there’s no evidence to support the notion of functional parallels between the biological dreaming process and the computational process of generative AI. She said that it’s important to understand that applications like ChatGPT are steady-state systems—in other words, they aren’t evolving and changing online, in real-time, even though they may be constantly refined offline.
“It’s not like [ChatGPT is] replaying and thinking and trying to combine things in new ways in order to cement what it knows or whatever kinds of things happen in the brain,” Pavlick said. “It’s more like: it’s done. This is the system. We call it a forward pass through the network—there’s no feedback from that. It’s not reflecting on what it just did and updating its ways.”
Pavlick said that when AI is asked to produce, for example, a rap song about the Krebs cycle, or a trippy image of someone’s dog, the output may seem impressively creative, but it’s actually just a mash-up of tasks the system has already been trained to do. Unlike a human language user, each output is not automatically changing each subsequent output, or reinforcing function, or working in the way that dreams are believed to work.
The caveats to any discussion of human intelligence or artificial intelligence, Serre and Pavlick emphasized, are that scientists still have a lot to learn about both systems. As for the hype about ChatGPT, specifically, and the success of neural networks in creating chatbots that are almost more human than human, Pavlick said it has been well-deserved, especially from a technological and engineering perspective.
“It’s very exciting!” she said. “We’ve wanted systems like this for a long time.”