AI and Twitter Could Help Predict Opioid Deaths
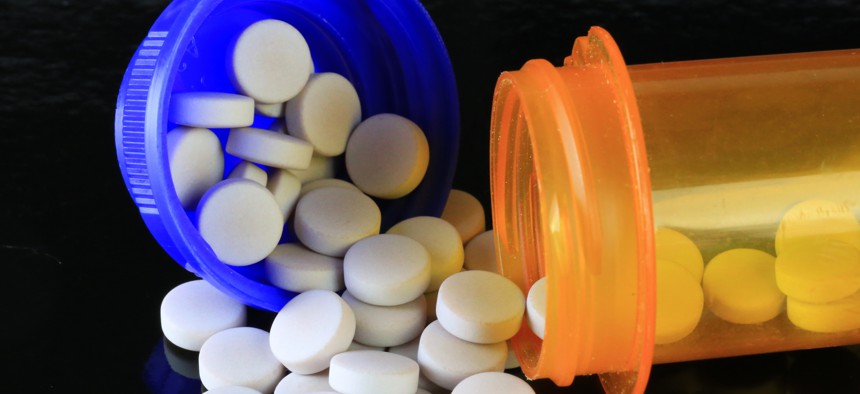
Douglas Sacha/Getty
Combined, the technologies can predict where opioid deaths are likely to occur.
A unique approach using artificial intelligence and social media posts could predict opioid mortality rates, researchers report.
The findings revealed that a sophisticated AI algorithm was able to predict opioid death rates—going back from previous years 2011 to 2017—much more accurately than using traditional information researchers and clinicians often use, such as prior rates in communities and socio-economic measures.
Clinicians and other professionals trying to stop the opioid crisis in the United States need all the tools they can to accurately identify communities at risk of large increases in deaths from opioid use and prevent deaths.
According to the National Center for Health Statistics, the US has been tackling an opioid epidemic for more than two decades. Age-adjusted opioid-related deaths have increased by some 350% over the 20 years of 1999 to 2020.
As reported in Nature Digital Medicine, the researchers created TrOP (Transformer for Opioid Prediction), a new model for community-specific trend projections that uses community-specific social media language, along with past opioid-related mortality data to predict future changes in opioid-related deaths.
According to the authors, TrOP builds on recent advances in sequence modeling, namely transformer networks, to use changes in yearly language on Twitter, as well as past mortality, to project the following year’s mortality rates in many US counties.
They used language data derived from the County Tweet Lexical Bank, a dataset that contains word usage on Twitter collected from more than 2,000 US counties starting from 2011. They built yearly topics or groupings that appeared together for each county from 2011 to 2017.
Using this language data as a central point, they combined it with data queried from the Centers for Disease Control and Prevention to gather the yearly opioid-related deaths per county. They then limited the full dataset to only US counties that reported opioid-related deaths for all of the years 2011 to 2017. This totaled data from 357 counties, which includes some 212 million people thus nearly two-thirds the population of the US.
This method predicted rates of opioid-related deaths that actually took place in the future with surprising accuracy.
“The main result of this study was in fact a statistical evaluation of how well our AI model’s predictions lined up with what really happened, and doing this without assuming any particular language used in social posts should equate to mortality,” says senior author H. Andrew Schwartz, associate professor in computer science at Stony Brook University.
The researchers found that the proposed model of predicting opioid-related deaths forecasted the actual yearly death rates within 1.15 deaths per 100,000 people, only a 3% absolute mean percent error.
Schwartz and his coauthors recognize the morbidity and mortality around opioid use and its prevention is a complex issue.
“Community-specific predictions are just one line of attack on what is a very complex problem that certainly needs a multifaceted and evolving response,” says Schwartz. “What this work with TrOP provides is the potential for more accurate predictions for opioid-related deaths, which would give professionals and community leaders the ability to better prepare and target areas where there is likely to be an increase in mortality.”
The researchers will continue to assess this AI model in different ways in order to test its accuracy. Given that social media and its wording continues to change and evolve, so to TrOP may have to be adjusted and updated in order to predict opioid-related deaths in communities across the country.
The authors suggest public health officials and those seeking to address the opioid epidemic try to deploy such an AI model to improve their existing community assessments to get a better idea of the direction of the epidemic in communities and regions in the coming year or years.
They also urge that any such algorithm using an AI model with social posts should include a replication of the empirical data findings and external validity checks, and that “all results should always be taken in consultation with other evidence of community opioid-related death risks.”