Future-proofing government data
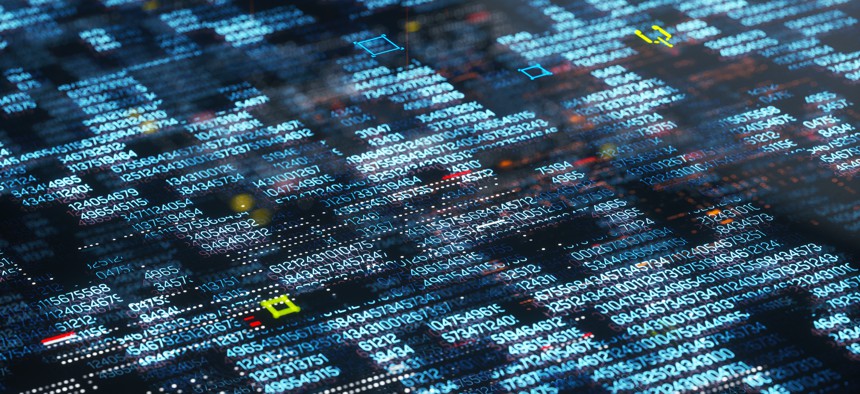
Andriy Onufriyenko/Getty Images
COMMENTARY: As AI becomes increasingly integrated into governmental operations, the cost of neglecting data readiness could be catastrophic.
Vast amounts of data are fueling innovation and decision-making, and agencies representing the United States government are custodian to some of the largest repositories of data in the world. As one of the world's largest data creators and consumers, the federal government has made substantial investments in sourcing, curating, and leveraging data across many domains. However, the increasing reliance on artificial intelligence to extract insights and drive efficiencies necessitates a strategic pivot: agencies must evolve data management practices to identify and discriminate synthetic data from organic sources to safeguard the integrity and utility of data assets.
AI's transformative potential is contingent on the availability of high-quality data. Data readiness includes attention to quality, accuracy, completeness, consistency, timeliness and relevance, at a minimum, and agencies are adopting robust data governance frameworks that enforce data quality standards at every stage of the data lifecycle. This includes implementing advanced data validation techniques, fostering a culture of data stewardship, and leveraging state-of-the-art tools for continuous data quality monitoring.
Synthetic data, artificially generated information that mimics real-world data, is a valuable resource for training AI models, especially in scenarios where actual data is scarce or sensitive. While synthetic data can augment organic datasets and be used to enhance model robustness, an overreliance on it may precipitate model collapse — a phenomenon where AI models fail to generalize and perform poorly in real-world applications. The risk is compounded when synthetic data is indistinguishable from organic data, potentially leading to skewed insights and flawed decision-making. To exacerbate the issue, pervasiveness of GenAI has made it easier to generate vast amounts of content quickly, outpacing traditional measures to identify and manage source material.
The ability to differentiate synthetic data from other sources is not just a technical challenge; it is a strategic imperative. Agencies must develop data structures and tagging protocols that clearly identify the provenance and nature of each data element. This metadata layer is essential for maintaining transparency, traceability, and trust in AI systems. It also serves as a safeguard against the inadvertent introduction of synthetic data biases into models that are intended to reflect real-world complexities. Metadata must be compatible with data science software, so that consumers of the data sources are able to easily reference and maintain lineage information when they leverage datasets for applications and analysis.
The investments made by government agencies in data acquisition and management are significant. As AI becomes increasingly integrated into governmental operations, the cost of neglecting data readiness and source differentiation could be catastrophic. Agencies must be proactive in managing these risks and invest in robust data architecture, rigorous data tagging standards, and continuous evaluation of the impact of synthetic data on AI model performance. By doing so, the US Government will protect their data investments and ensure that AI systems are built on a foundation of integrity and representativeness, serving the public good with greater efficacy and reliability. The time to act is now; the future of government data depends on it.
NEXT STORY: Why unified observability is key to better UX