How to be data-ready for AI adoption
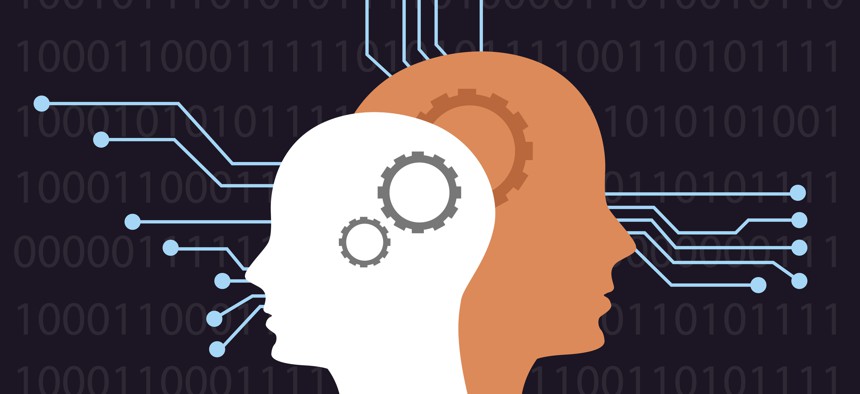
ribkhan/Getty Images
COMMENTARY: Outcomes are only as reliable as the data supporting generative AI tools.
As more public entities embark on utilizing generative artificial intelligence, it is crucial to ensure that the data being used is AI-ready as there are several specific threats to data protection when implementing Gen AI initiatives. Let’s dive further into the key elements to consider.
The first is data quality. Data is the lynchpin of effective and responsible use of GenAI, highlighting the need for using clean, vetted and current data to receive accurate and relevant responses from GenAI applications. Many GenAI platforms could accidentally access irrelevant or out of date data via a poorly managed data system or could collect a large amount of ‘junk’ data, which can influence the insights and responses GenAI delivers. If these types of data are used then it could lead to inaccurate or wrong outputs – along the lines of “garbage in, garbage out.”
In a world where large language models can be trained on publicly available digital data — mainly uncontrolled data from the internet — the value of trusted datasets grows exponentially when underpinned by validated, historical and reliable data. It is more important than ever to get your data strategy right. For government agencies, data quality is a huge concern especially when flipping the switch on AI initiatives. Agencies need the insight, direction and confidence that only comes with current and actionable data as the quality of data you put in directly impacts the quality of the data you get out.
It is essential to have data that is GenAI-ready and helps prevent data “Frankenstein.” Inaccurate data used as data inputs could also lead to hallucinations, or instances where GenAI models confidently return an answer that appears correct but is actually wrong. Hallucinations are becoming more of a pervasive issue as organizations incorporate GenAI.
Another key element for creating GenAI-ready data is regarding data hygiene and ensuring that clean, accurate, updated and high-quality data is being used. If an organization has poor data hygiene, then there could be serious issues.
Data needs to be high quality without structural errors or duplications. As outcomes are only as good as the data put in, agencies want to know what data sets were involved in creating its GenAI outputs to establish data provenance, or a documented trail that accounts for the origin and changes of a piece of data. This helps provide the ability for a user to trace and validate the data sourcing that led to the answer. Having a clear trail that shows what data sets were used in the GenAI outputs can also show if and where hallucinations occur.
Organizations are facing more threats related to data protection such as data poisoning or prompt injections that can influence model results and outcomes. Also of concern is model theft, where a bad actor reverse engineers a business's Gen AI model that could lead to a loss of intellectual property and data ownership.
Due to these ongoing threats, there is growing prominence of chief data officer roles in government. The role of a CDO can be very effective when it comes to AI experimentation with someone who can best understand the quality and type of data being used for Gen AI applications. Public agencies need to put effort and investment into ensuring that data is accurate and timely and controlling data sources tightly so that the right data is going into their respective data supply chains.
Lastly, having an adequate data governance system in place is critical for implementing GenAI. There are essential elements of a data governance system, including robust data classification, categorization, and data provenance, as well as data quality assurance and data minimization practices. These types of controls facilitate consistency and clear guidance around data integrity, who holds what responsibilities, and how information is protected across the data supply chain and handled in compliance with laws and regulations, among other things.
Having AI-ready data will allow organizations to take advantage of and unlock the true potential of GenAI. Getting data AI-ready is essential for maximizing the effectiveness of AI technologies and ensuring accurate, reliable and effective outcomes — which are absolutely critical for any public or private entity.