What happens when machines break the law?
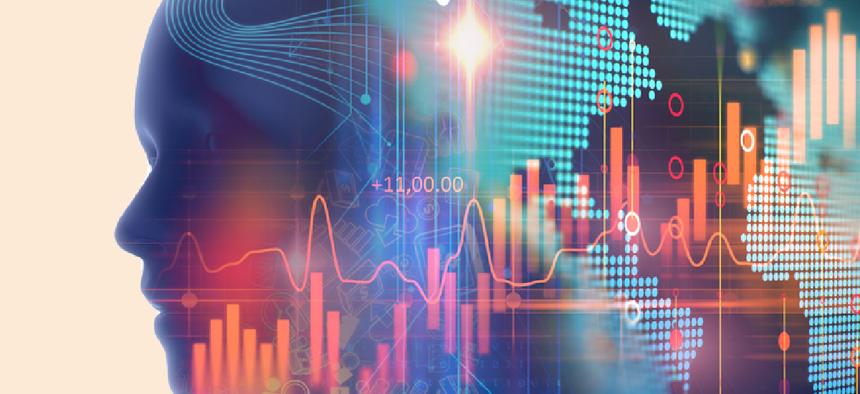
Governments, private companies and financial institutions are all using AI to automate simple and complex processes. But what happens when an algorithm breaks the law and humans can't explain why?

In ever greater numbers, governments, private companies and financial institutions are using algorithms to automate both simple and complex processes. But what happens when an algorithm breaks the law and the humans running the program aren't aware?
The question is becoming more relevant as organizations increasingly rely on algorithms to trade stocks, target advertisements and conduct financial lending and other transaction -- in some cases with limited insight into how those decisions are made.
Nicol Turner-Lee, a fellow at the Center for Technology Innovation at the Brookings Institution, told lawmakers at a June 26 House Financial Services Committee that the explosion of data has allowed for development of more sophisticated algorithms that can make inferences about people -- from their identities and demographic attributes to their preferences and likely future behaviors.
But algorithms also compare people and things to related objects, identify patterns and make predictions based on those patterns, and that's where bad data or flawed learning protocols can cross the line. A study by the University of Berkeley last year found that online automated consumer lending systems charged black and Latino borrowers more to purchase and refinance mortgages than white borrowers, resulting in hundreds of millions of dollars of collective overcharging that couldn't be explained.
"We are seeing people being denied credit due to the factoring of digital composite profiles, which include their web browsing histories, social media profiles and other inferential characteristics, in the factoring of credit models," Turner-Lee said. "These biases are systematically [less favorable] to individuals within particular groups when there is no relevant difference between those groups which justifies those harms."
Technology is not necessarily the main culprit here, and the Berkeley study did find that in some cases financial technology, or fintech, algorithms can actually discriminate less than their human lender counterparts. Rather, most experts say it's the humans and organizations behind those flawed algorithms that are careless, feeding data points into a system without having a real understanding of how the algorithm will process and relate that data to groups of people. However, the speed and scale at which these automated systems operate mean they have the potential to nationally spread discriminatory practices that were once local and confined to certain geographic enclaves.
While organizations are ultimately legally responsible for the ways their products, including algorithms, behave, many encounter what is known as the "black box" problem: situations where the decisions made by a machine learning algorithm become more opaque to human managers over time as it takes in more data and makes increasingly complex inferences. The challenge has led experts to champion "explainability" as a key factor for regulators to assess the ethical and legal use of algorithms, essentially being able to demonstrate that an organization has insight into what information its algorithm is using to arrive at the conclusions it spits out.
The Algorithmic Accountability Act introduced in April by Sens. Cory Booker (D-N.J.) and Ron Wyden (D-Ore.) in the Senate and Rep. Yvette Clarke (D-N.Y.) in the House would give the Federal Trade Commission two years to develop regulations requiring large companies to conduct automated decision system impact assessments of their algorithms and treat discrimination resulting from those decisions as "unfair or deceptive acts and practices," opening those firms up to civil lawsuits. The assessments would look at training data for impacts on accuracy, bias, discrimination, privacy and security and require companies to correct any discrepancies they find along the way.
In a statement introducing the bill, Booker drew on his parents' experience of housing discrimination at the hands of real estate agents in the 1960s, saying that algorithms have the potential to bring about the same injustice but at scale and out of sight.
"The discrimination that my family faced in 1969 can be significantly harder to detect in 2019: houses that you never know are for sale, job opportunities that never present themselves, and financing that you never become aware of -- all due to biased algorithms," he said.
Turner-Lee said that organizations can do more to understand how their automated systems may be susceptible to illegal or discriminatory practices during the design stage and before they're deployed. Voluntary or statutory third-party audits and bias-impact statements could help companies to "figure out how to get ahead of this game," she said.
"Getting companies as well as consumers engaged, creating more feedback loops, so that we actually go into this together, I think, is a much more proactive approach than trying to figure out ways to clean up the mess and the chaos at the end," Turner-Lee said.
Federal agencies are also increasingly making use of artificial intelligence and machine learning and will face many of the same conundrums. Another bill sponsored by Sens. Cory Gardner (R-Colo.), Rob Portman (R-Ohio), Kamala Harris (D-Calif.) and Brian Schatz (D-Hawaii) would create a new Center of Excellence at the General Services Administration to provide research and technical expertise on AI policy. It would also establish a federal advisory board to explore opportunities and challenges in AI and require agencies to create governance plans for how use of the technology aligns with civil liberties, privacy and civil rights.