Can AI decide if work is 'inherently governmental?'
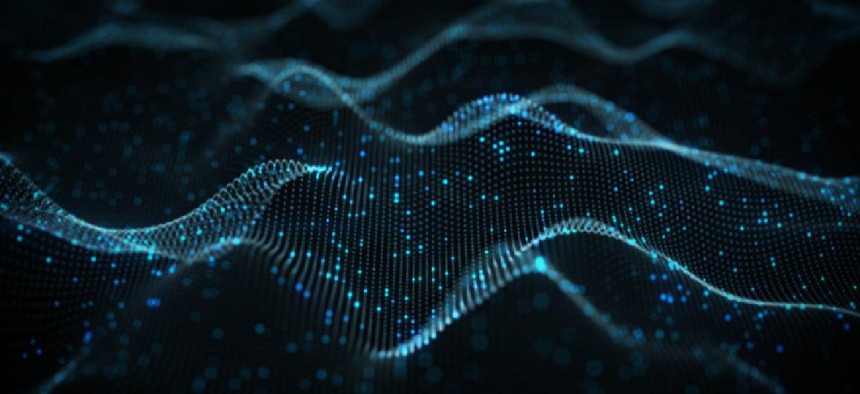
The Department of Health and Human Services is using a recurrent network to get smarter about its acquisition efforts.

Determining whether a function is inherently government is a critical step when an agency is procuring services, and it's harder than one might think. So the Department of Health and Human Services has built a recurrent neural network to help acquisition staff make the call.
The effort is still in proof-of-concept stage, HHS CIO Jose Arrieta told FCW, but the artificial intelligence-powered system already is making the correct determination 86% of the time. The goal is not to automate the entire process, he said, but rather to allow HHS' human experts to focus on the toughest and most-borderline cases.
Those same experts were used to help train the neural network, which used some 9,000 HHS statements of work from past procurements and the Government Accountability Office's guidelines on inherently governmental functions as the starting point.
"What we're trying to do is provide insight so that someone that's really smart can look at what we did and they can say … 'That's not the right prediction,'" Arrieta said. "And that's fine. Or they come back and say, 'You know what, that actually really helped.'"
The pilot project was originally conceived as a "Turbo Tax-style" tool to help contracting officer write new solicitations by pulling appropriate language from past examples, Arrieta said, but Assistant Secretary for Administration Scott Rowell suggested the focus on whether solicitations were touching on inherently governmental functions.
The project took less than six weeks to spin up, Arrieta said, but it did require a different way of thinking. First, there was the need for a recurrent neural network, which can pass outputs back through the system for additional analysis and are especially well-suited to natural language processing. Then all that text from 9,000 solicitations had to be scored.
"You can't do predictive analytics or any type of deep learning on text," Arrieta said. "You have to do it on a number. … We're scoring the level of importance of the words -- how many times is a word repeated?... And then we take that number string that we developed, that vector, and we push it into a predictive model."
The total cost to date has been less than $300,000, he said, and the project identified roughly $100 million in potential savings just on the initial batch of statements of work – 11% to 12% of the total portfolio value. But the goals are bigger than just cost savings, Arrieta argued.
"The better you understand when you need federal employees and when you need contractors to perform a service, the better service outcome you will provide," he said. In addition to the tasks that must by law be performed by federal employees, there are some where government workers would be cheaper than contractors, he noted. Other times outsourcing brings clear cost savings, or "the need is related to a business line that you're standing up, but you don't know if it will actually work," he said. "This type of insight allows us to shape our business model in a way that better serves the customer," Arrieta said.
Other uses of recurrent neural networks are already on the drawing board at HHS. A small cybersecurity pilot -- where HHS found it already had access to the neural network via an existing contract -- is underway, and Arrieta's team intends to tease new acquisition insights out of the solicitation data.
"We also looked at the labor categories," which could help to negotiate better prices for future services contracts, he said. "Can you start to use that same type of analysis to look at job descriptions and start to get an understanding of the types of folks that may apply … so that you can fine tune that job description in a way to truly get the type of candidate you're looking for?"
"We think the lessons learned here are applied on a much broader," he said, "and can be applied to other problems."